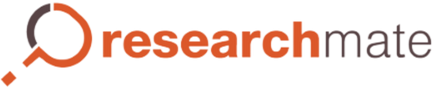

Effective Research Analysis: Essential Methods, Tools, and 6 Key Challenges
Introduction.
Research is a fundamental aspect of human knowledge and progress, serving as the backbone for innovation, policy-making, and scientific discovery. In an era shaped by the Industrial Revolution IR 4.0 —where technologies like big data , artificial intelligence , and IoT drive unprecedented data generation—understanding the nuances of research becomes increasingly vital.
This article aims to provide a comprehensive review of research analysis, shedding light on their definition, significance, tools, and real-world applications. By delving into the intricacies of research, we can better appreciate its role in shaping our understanding of complex issues and driving informed decision-making.
Meaning of Research Analysis
Research analysis is a critical component of the research process , serving as the bridge between data collection and the extraction of meaningful insights. It involves evaluating and interpreting the information gathered during research to make informed decisions and conclusions. This process is not merely about presenting data; it requires a systematic approach to dissecting the information, identifying patterns, and understanding the implications of the findings. By breaking down complex data into manageable parts, researchers can gain a clearer understanding of the subject matter and communicate their results effectively.
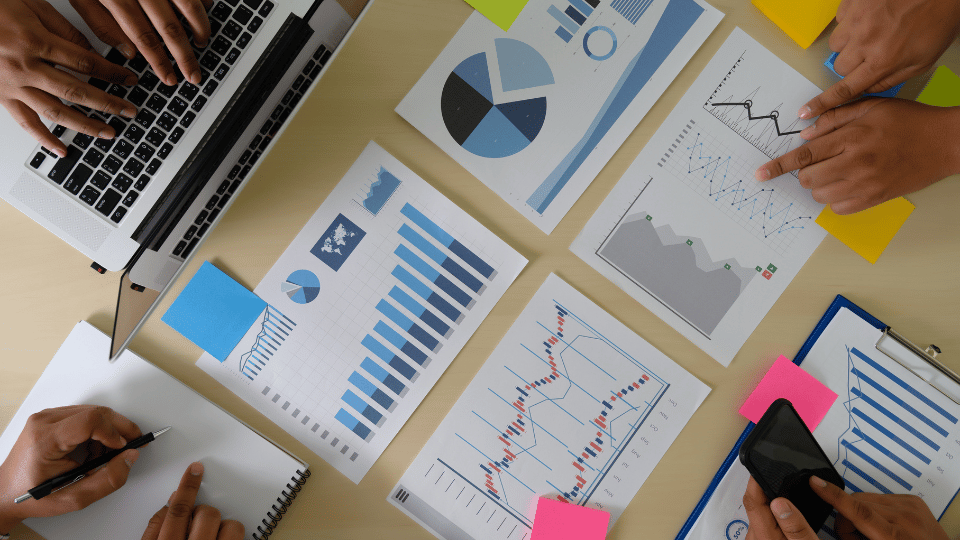
At its core, research analysis transforms raw data into actionable knowledge. This transformation involves several steps, including data cleaning, modeling, and interpretation, which collectively aim to uncover trends and relationships within the data. The significance of research analysis lies in its ability to provide clarity and context to the findings, allowing researchers to draw conclusions that are not only valid but also relevant to the field of study. Ultimately, effective research analysis empowers researchers to contribute valuable insights that can influence decision-making and drive advancements in various disciplines.
Categories of Research Analysis Methods
Research analysis methods can be broadly categorized into two main types: qualitative and quantitative analysis. Qualitative analysis focuses on understanding underlying reasons, opinions, and motivations, often utilizing qualitative research instruments such as interviews , focus groups, and open-ended surveys. In contrast, quantitative analysis emphasizes numerical data and statistical techniques, employing methods like experiments, structured surveys, and observational studies to quantify variables and identify patterns.
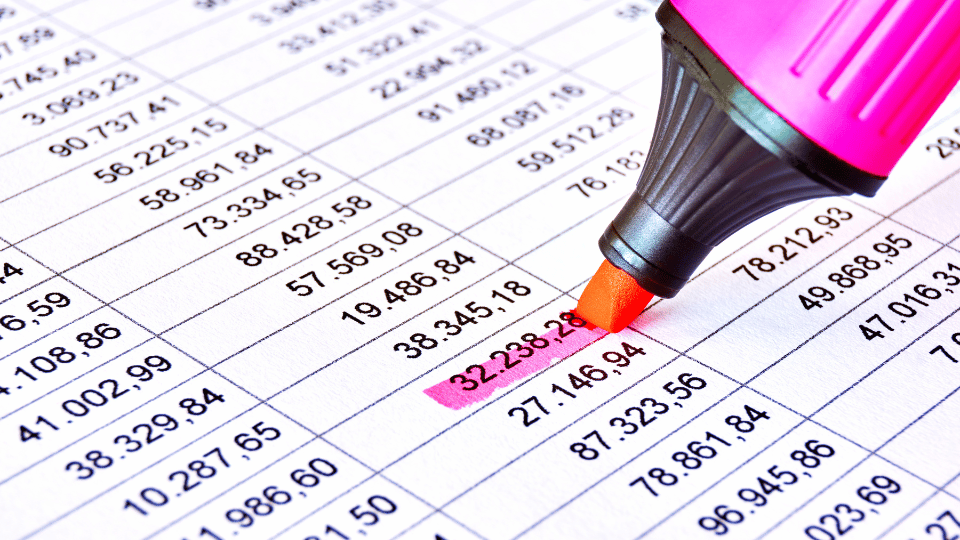
The choice of research analysis method significantly impacts the outcomes of a study. Qualitative methods are particularly useful for exploratory research , allowing researchers to delve deep into complex issues and gather rich, detailed information. However, they can be subjective and may not always be generalizable.
On the other hand, quantitative methods provide a more objective framework, enabling researchers to test hypotheses and make predictions based on statistical evidence. Yet, they may overlook the nuances of human behavior and context. Understanding the strengths and limitations of each method is crucial for researchers to select the most appropriate approach for their specific research questions .
Tools To Carry Out Research Analysis
For qualitative research , software like NVivo, Atlas.ti, and Looppanel are invaluable, as they assist researchers in coding and analyzing unstructured data. These tools enable the identification of patterns and themes, transforming raw data into structured insights that can inform decision-making and further research.
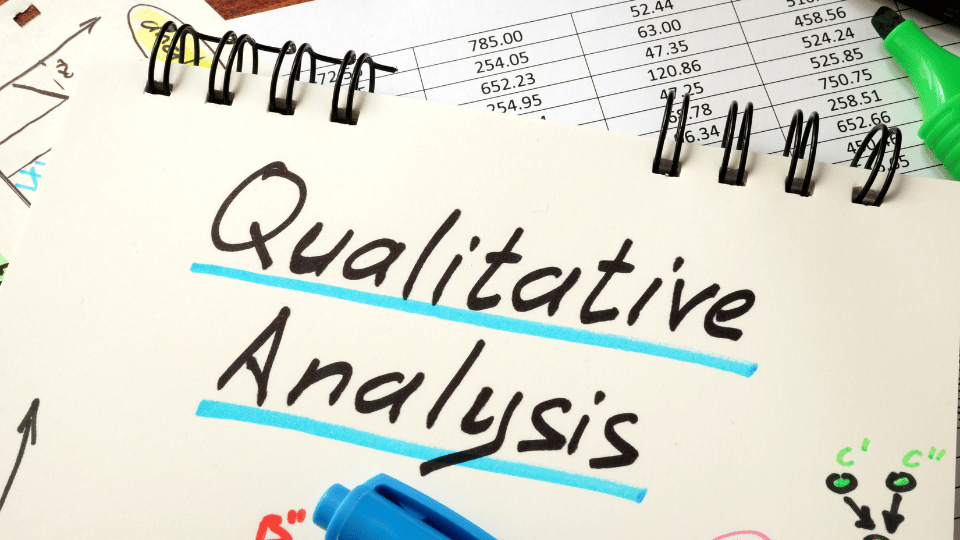
For quantitative research , the landscape is equally diverse, ranging from basic spreadsheet applications to advanced statistical software. Tools such as SPSS, R, and Python libraries provide researchers with the capability to perform complex statistical analyses, ensuring that data is interpreted accurately. The choice of tool often depends on the specific requirements of the research project, including the type of data being analyzed and the desired outcomes.
6 Challenges in Research Analysis
Securing adequate funding.
One of the most significant challenges in research analysis is obtaining sufficient funding, as grants are often highly competitive. Limited financial resources can restrict the scope and depth of research projects, impacting both the quality and quantity of data collected.
High Costs and Time Constraints in Execution
Research studies are often slow and costly, which can create frustration for researchers, particularly when working within tight timelines. The expenses associated with extensive data collection and analysis can delay progress and hinder timely results.
Inconsistencies Between Research Questions and Methodologies
A frequent issue in research analysis is the misalignment between research questions and methodologies . When the chosen methods do not fully align with the objectives, it becomes challenging to draw valid conclusions, leading to confusion and potentially unreliable results.
Data Discrepancies in Secondary Research
Data discrepancies across different sources present a common obstacle, especially in secondary research. Variations in data collection methods , sample sizes, or demographics complicate analysis, making it difficult to maintain consistency and accuracy in findings.
Limited Collaboration and Isolation Among Researchers
Researchers often work in isolation or within departmental silos, limiting collaboration and cross-disciplinary insights. This lack of communication can result in a fragmented understanding of the research topic , as shared knowledge and diverse perspectives are essential for comprehensive analysis.
Challenges in Qualitative Data Analysis
Qualitative data analysis presents unique difficulties, including the need for rigorous methodology and the potential for researcher bias. Without careful handling, these challenges can compromise the credibility and reliability of qualitative research outcomes.
In conclusion, research forms the foundation of knowledge creation and innovation across diverse fields, offering a systematic approach to understanding complex phenomena and informing decisions. Its importance is evident in the progress made in science, technology, and social sciences, all of which contribute to societal advancement. Effective research analysis is essential for drawing meaningful insights from data, providing a basis for reliable, impactful findings that drive these advancements forward.
However, the research journey presents numerous challenges, from question formulation to choosing the right analysis methods and tools. As discussed, navigating these obstacles requires a careful balance of best practices and adaptability to maintain the integrity of findings . By refining their approaches and staying aware of potential pitfalls, researchers can enhance the rigor and quality of their work, ultimately fostering a culture of inquiry and continuous innovation.
Leave a Comment Cancel reply
Save my name, email, and website in this browser for the next time I comment.
Related articles
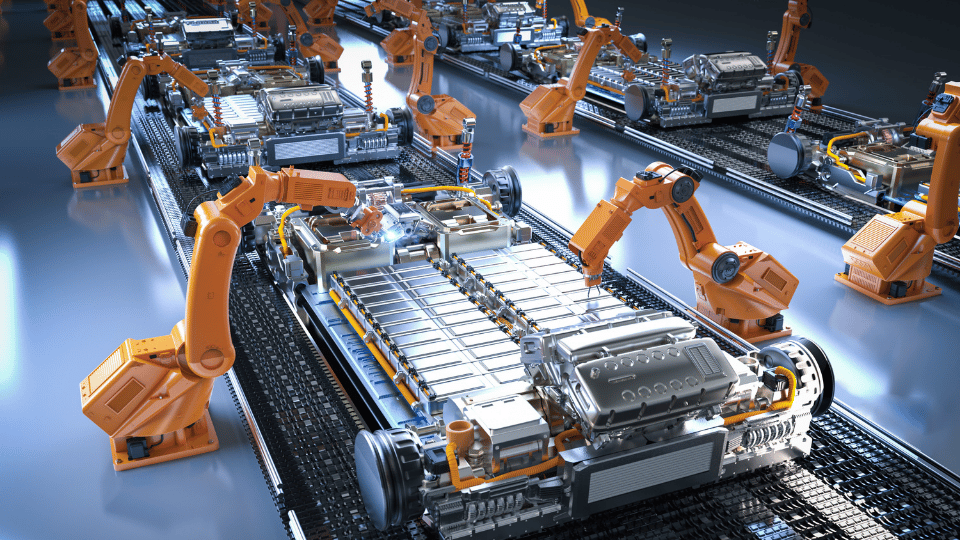
6 Breakthrough Insights of Reinforcement Learning Algorithms
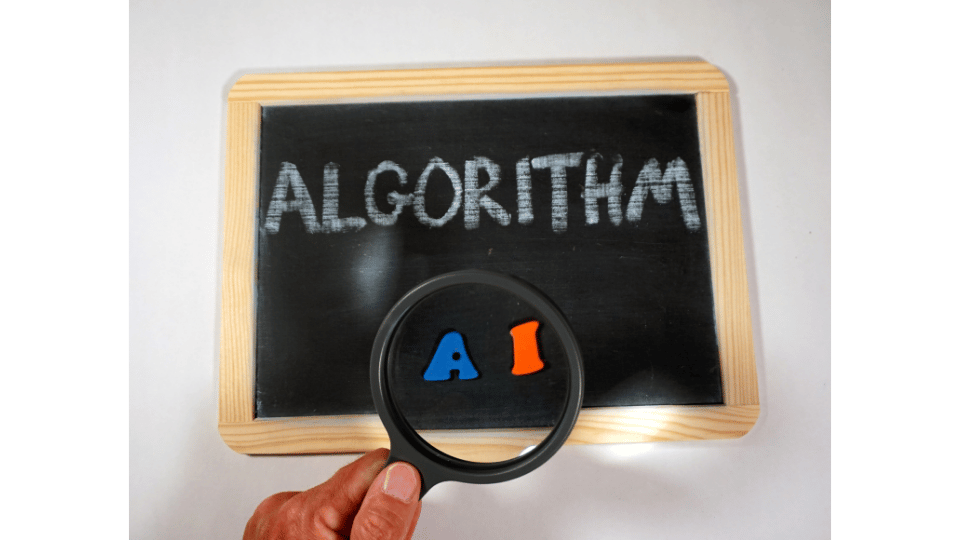
7 Breakthrough Insights of Unsupervised Learning Algorithms
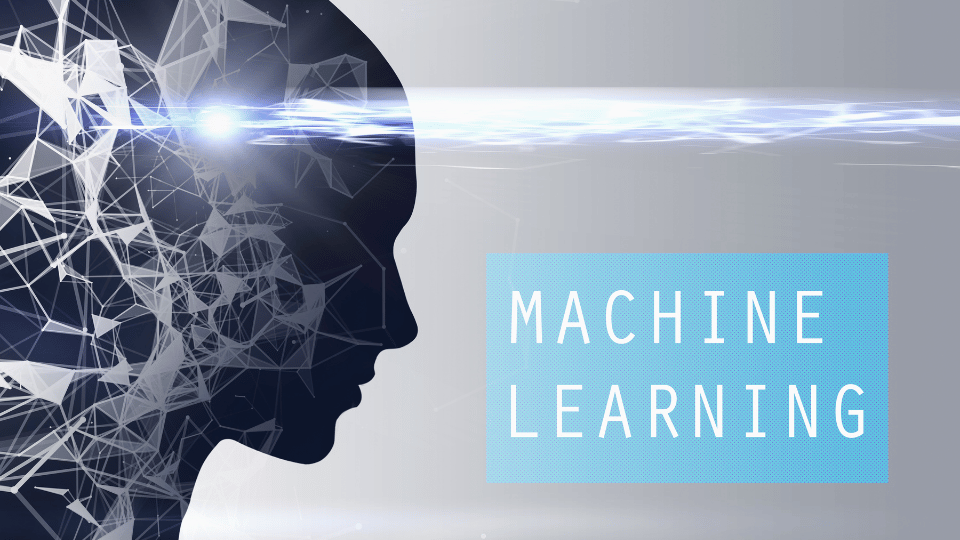
8 Best Insights of Supervised Learning Algorithms Unveiled
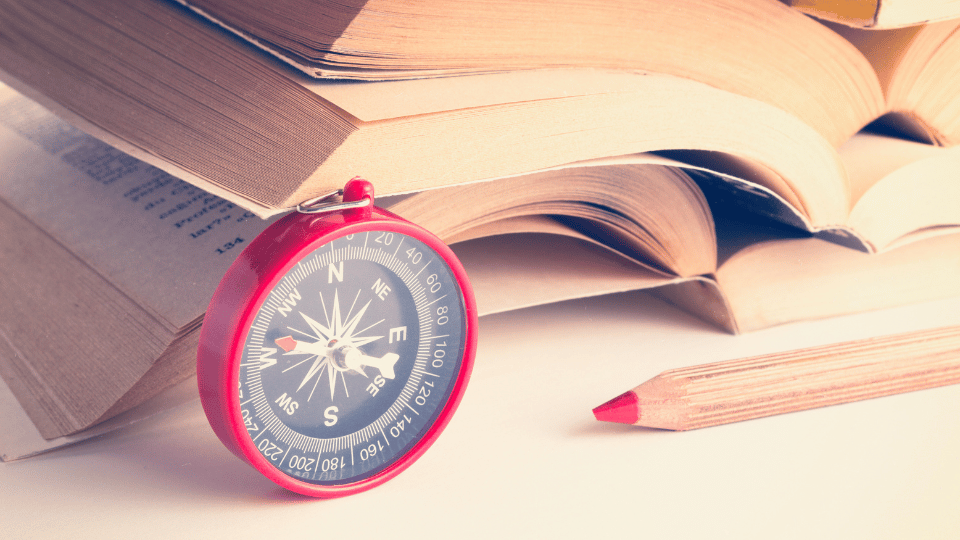
Defining Your Research Interest: 4 Essential Tips!
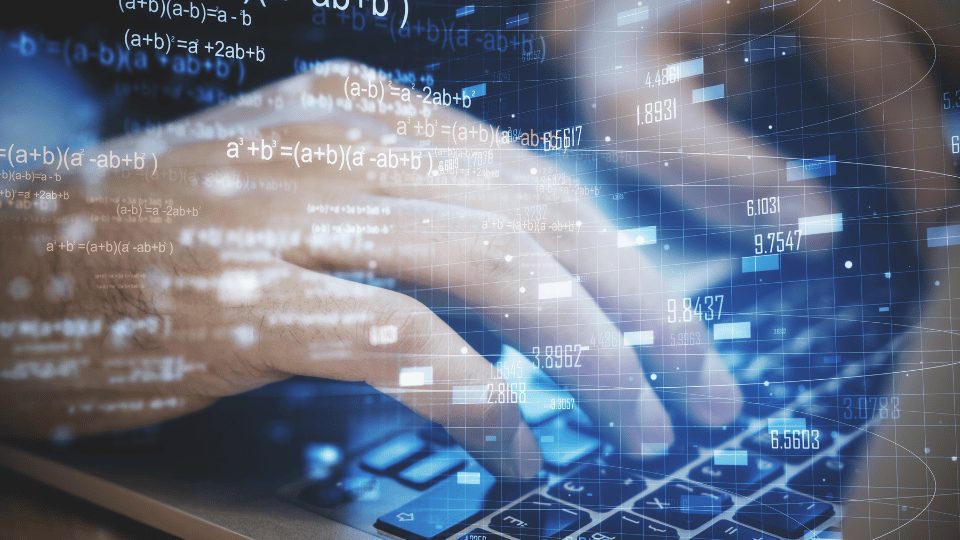
Significance of Quantitative Research : 9 Essential Insights
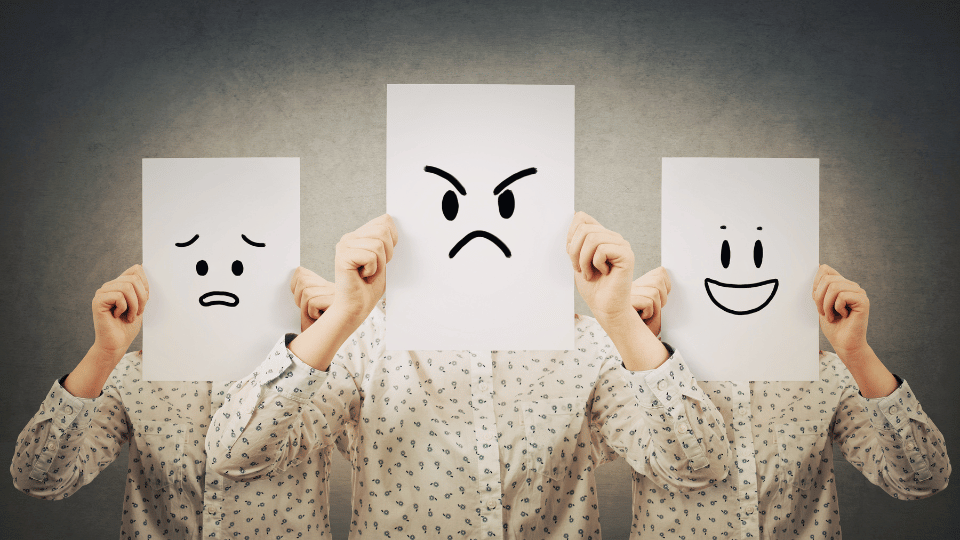
Qualitative Research: Valuable Insights for In-Depth Understanding
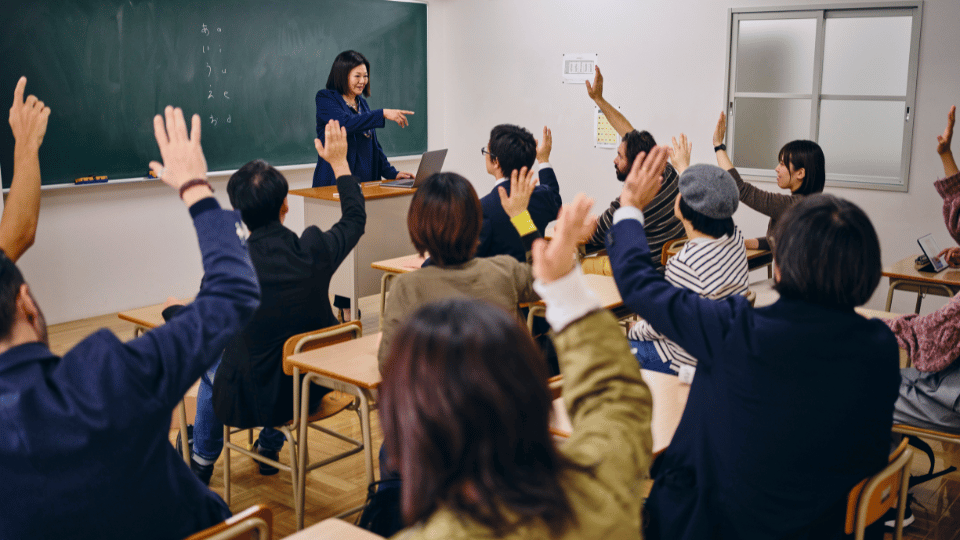
Causal-Comparative Research : 8 Crucial Insights To Success
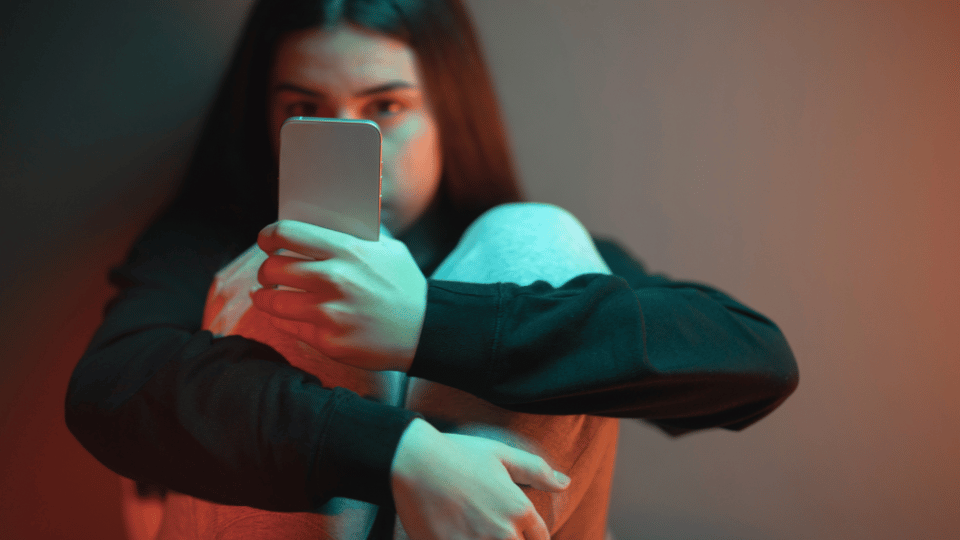
Correlational Research: Valuable and Essential Insights!
Research Analytics: Driving Innovation and Efficiency in Research Management
Updated on Nov 20th, 2024
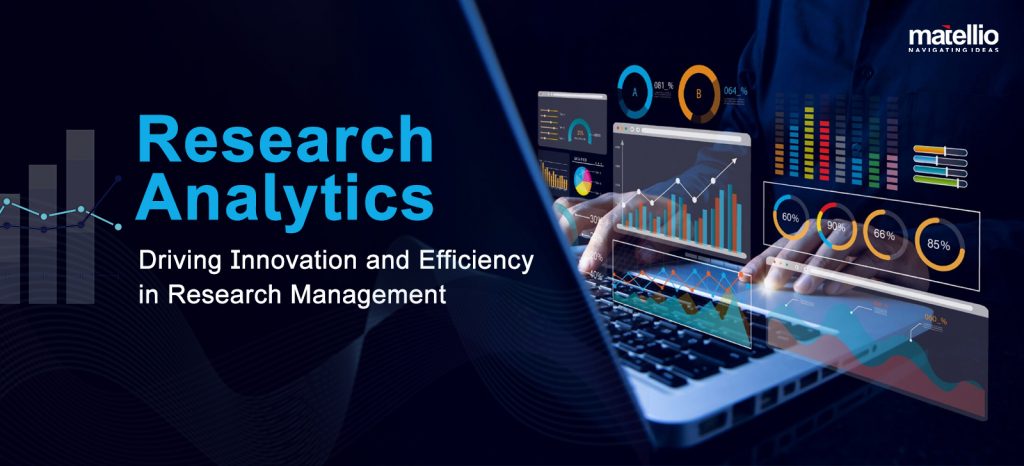
In today’s rapidly evolving research landscape, the role of data-driven insights has become paramount . Research analytics refers to the systematic application of data analysis techniques to derive actionable insights from various research activities. This process involves utilizing sophisticated statistical methods, data management tools, and visualization techniques to transform raw data into meaningful information.
Moreover, it fosters collaboration among researchers, facilitating knowledge sharing and interdisciplinary partnerships. In an era where research problems are often complex and multifaceted, collaborative efforts have become essential for addressing challenges and driving innovation.
- The core components of research analytics include data collection, data management, statistical analysis, visualization, and reporting.
- The benefits of research analytics include enhanced decision-making, increased efficiency, improved collaboration, higher quality research, real-time monitoring, informed funding decisions, and greater innovation.
- Matellio’s research grant process automation project streamlined the process and improved efficiency, transparency, and stakeholder collaboration.
- Challenges in research analytics include data quality, integration issues, resistance to change, data privacy concerns, and skill gaps, which can be addressed through data validation, data integration, training, data governance, and expertise development.
- Future trends in research analytics include AI-driven insights, open science initiatives, automated data analysis, interdisciplinary research, and ethical data usage. Future trends in research analytics include AI-driven insights, open science initiatives, computerized data analysis, interdisciplinary research, and ethical data usage.
Table of Contents
Key Components of Research Analytics
Research analytics encompasses several critical components that collectively enhance the effectiveness of research activities. Each component plays a vital role in transforming raw data into actionable insights, ultimately improving the overall research process.
Data Collection
Data collection is the foundational step in research analytics, involving the systematic gathering of diverse data from various sources. This can include:
- Experiments : Collecting quantitative and qualitative data from controlled experiments to test hypotheses.
- Surveys : Designing and distributing surveys to gather opinions, behaviors, and preferences from target populations.
- Literature Reviews : Aggregating existing research findings and data from scholarly articles, books, and other academic sources to build a comprehensive understanding of the topic.
Effective data collection ensures that researchers have a robust dataset to analyze, which is essential for drawing valid conclusions and insights.
Data Management
Once data is collected, it must be organized and stored effectively to facilitate easy access and retrieval. Key aspects of data management include:
- Data Organization : Structuring data in databases or data warehouses that allow for efficient storage and querying.
- Data Cleaning : Identifying and correcting errors, inconsistencies, and missing values in the dataset to ensure accuracy.
- Data Governance : Establishing policies and procedures to manage data quality, security, and compliance with regulations (e.g., GDPR).
Proper data management practices are crucial for maintaining the integrity of the dataset and ensuring that researchers can easily access the information they need.
Statistical Analysis
Statistical analysis involves applying various statistical techniques to interpret data and extract meaningful patterns. This component includes:
- Descriptive Statistics : Summarizing and describing the main features of a dataset, such as mean, median, and standard deviation.
- Inferential Statistics : Using sample data to make generalizations about a population, employing techniques like hypothesis testing and confidence intervals.
- Regression Analysis : Identifying relationships between variables and predicting outcomes based on observed data.
Statistical analysis enables researchers to derive insights from data, identify trends, and make informed decisions based on empirical evidence.
Visualization
Data visualization is presenting data insights through graphical representations like graphs, charts, and dashboards. Effective visualization techniques include:
- Charts and Graphs : Bar charts, line graphs, scatter plots, and pie charts are used to represent data visually, making it easier to understand patterns and relationships.
- Dashboards : Creating interactive dashboards that consolidate key metrics and insights in one place, allowing for real-time monitoring and analysis.
- Infographics : Developing visual representations of complex data to communicate findings clearly and engagingly.
By utilizing visualization tools, researchers can convey their findings more effectively to stakeholders, enhancing comprehension and engagement.
The final component of research and analytics involves compiling analytical findings into comprehensive reports that inform stakeholders and decision-makers. Key elements of effective reporting include:
- Structured Format : Organizing reports in a clear and logical structure, including an executive summary, methodology, findings, and conclusions.
- Actionable Insights : Highlighting key takeaways and recommendations based on the analysis to guide decision-making processes.
- Stakeholder Engagement : Tailoring reports to meet the needs and interests of various stakeholders, such as funding agencies, research teams, and policymakers.
Effective reporting ensures that research findings are communicated clearly and can be utilized to inform future research directions, policy decisions, and strategic planning.
Read More: Analytics as a Service: Revolutionizing Data-Driven Decision Making for Modern Businesses
Benefits of Implementing Research Analytics
Implementing analytical research offers numerous unique advantages that significantly enhance the overall research process. These benefits extend beyond individual projects, positively impacting research teams, funding agencies, and the broader research community. With the suppo rt of technology consulting services , businesses can optimize their research processes, ensuring the integration of advanced tools and methodologies that drive better insights and more efficient outcomes.
Enhanced Decision-Making
Data-driven insights derived from analytic research empower researchers to make informed decisions at every stage of their projects. Researchers can develop hypotheses and choose methodologies that are backed by evidence after analyzing the trends. This leads to more effective strategies, higher-quality results, and better alignment with research objectives. Additionally, stakeholders can make strategic decisions based on comprehensive data analyses, ensuring resources are allocated to the most promising projects.
Increased Efficiency
The integration of research analytics streamlines various stages of the research process, significantly enhancing operational efficiency. Automated data collection and processing reduces the time spent on manual tasks, allowing researchers to focus on analysis and interpretation. This efficiency extends to the entire research lifecycle, from initial data gathering to final reporting, ultimately leading to faster project completion and delivery of results.
Improved Collaboration
Research analytics fosters a collaborative environment among research teams by providing tools and platforms that facilitate communication and knowledge sharing. Analytics tools often feature collaborative functionalities, allowing team members to work together in real-time, share insights, and provide feedback. This collaboration not only enhances the quality of research but also encourages the cross-pollination of ideas, leading to more innovative solutions.
Higher Quality Research
Access to robust data and advanced analytical techniques significantly improves the quality and reliability of research findings. Researchers can conduct thorough analyses, validate results, and support their conclusions with solid evidence. The use of analytics helps to identify anomalies in data that may require further investigation, ensuring that research outputs are accurate and trustworthy. This focus on quality ultimately leads to stronger contributions to the field.
Real-Time Monitoring
Analytic research enables real-time monitoring of projects, allowing researchers to track progress and adjust as necessary. This capability is especially valuable in dynamic research environments where conditions may change rapidly. By having access to real-time data, researchers can quickly identify issues or challenges, allowing them to pivot their strategies to stay on track. This agility is essential for maintaining project momentum and achieving desired outcomes.
Informed Funding Decisions
For funding agencies, research analytics provides valuable insights into project feasibility, impact, and alignment with funding priorities. By analyzing data related to past projects and their outcomes, agencies can make informed decisions about which proposals to support. The data-driven approach helps to ensure that projects have the best resource allocation with the highest potential for success and societal benefit, fostering a more effective funding landscape.
Greater Innovation
By identifying trends, gaps, and emerging areas of interest in existing research, analytics fosters greater innovation within the research community. Researchers can leverage data to explore new hypotheses, address unmet needs, and develop cutting-edge solutions. This proactive approach not only advances individual research projects but also contributes to the overall progression of knowledge across disciplines.
Transform Your Research Data into Actionable Strategies!
Connect With Our Experts!
What is 1 + 5 =
Matellio’s Expertise in Research Analytics
Matellio has established itself as a leader in research analytics, offering tailored solutions that significantly enhance research management processes across various domains. With a keen focus on integrating advanced technologies, Matellio empowers researchers and institutions to leverage data for better decision-making and efficient project execution.
A prime example of Matellio’s impactful contributions is the research grant process automation project, designed to address the intricate challenges associated with managing research grants. Recognizing that the grant application and approval process can often be lengthy and cumbersome, Matellio set out to develop a solution that streamlines these workflows.
By automating key processes and providing a centralized platform for managing grant applications, our solution has significantly improved efficiency, transparency, and collaboration among stakeholders. The project exemplifies Matellio’s commitment to harnessing digital transformation services for the betterment of research practices, ensuring that researchers can focus more on innovation and less on administrative hurdles.
Overview of the Project and Its Objectives
The Research Grant Process Automation project aimed to simplify the complexities of research grant management, which often involves multiple stakeholders and intricate procedures. The primary objectives were to:
- Reduce the time taken for grant applications to be reviewed and approved.
- Enhance communication between researchers, grant administrators, and funding bodies.
- Increase transparency in the grant application process, allowing stakeholders to track application statuses in real-time.
How Matellio’s Solution Streamlined Research Grant Processes?
Matellio’s innovative approach involved the implementation of a centralized grant management platform. This platform enabled seamless communication between researchers and grant administrators, facilitating:
- Automated Notifications : Stakeholders receive real-time updates about application statuses, deadlines, and required documentation, minimizing confusion and delays.
- Streamlined Workflows : By automating repetitive tasks and approvals, the platform significantly cuts down processing times and reduces the risk of human error.
- Data-Driven Insights : The platform provides analytics capabilities that allow administrators to assess grant application trends, identify bottlenecks, and make informed decisions to improve the process.
The result is a more efficient grant management system. It accelerates the approval process and fosters a collaborative environment where researchers can focus on their projects rather than administrative burdens.
Relevance of This Project to Broader Research Analytics Goals
The research grant process automation project aligns seamlessly with broader research analytics goals by:
- Enhancing Operational Efficiency : The reduction in processing times and automation of workflows lead to faster project initiation and execution, enabling researchers to bring their ideas to fruition more quickly.
- Ensuring Transparency : By providing stakeholders with real-time visibility into application statuses and processes, the solution cultivates trust and accountability within the research community.
- Fostering Innovation : By alleviating administrative pressures, researchers can dedicate more time and resources to innovative research activities, ultimately contributing to advancements in their respective fields.
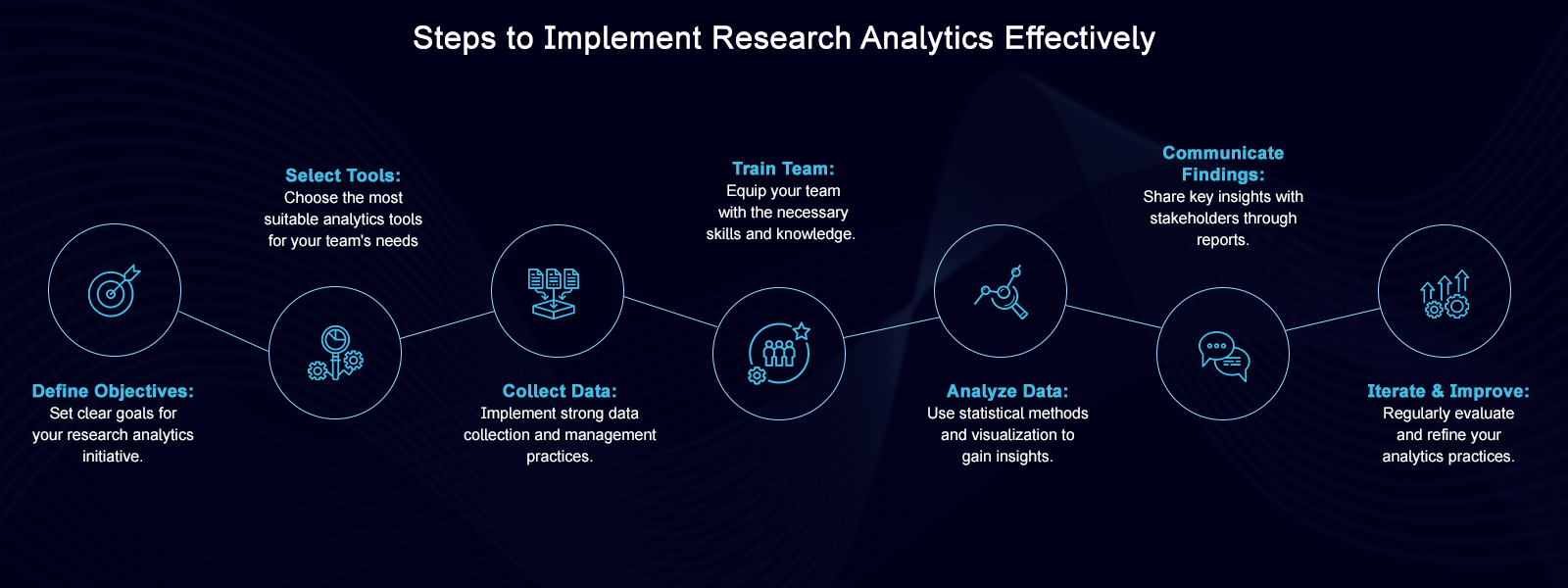
Challenges and Solutions in Research Analytics
Despite its advantages, research analytics faces several unique challenges that can hinder effective implementation and outcomes. Addressing these challenges requires targeted solutions to ensure the successful integration of analytics in research processes:
Data Quality Issues
Inaccurate, incomplete, or inconsistent data can lead to flawed analyses and misinformed decisions, undermining the credibility of research findings.
Solution : Implement robust data validation and cleaning processes before analysis. This involves establishing protocols for data entry, performing regular audits, and using automated tools to identify and rectify discrepancies. By ensuring high data quality, researchers can enhance the reliability of their analyses.
Integration of Diverse Data Sources
Combining data from various platforms, such as surveys, databases, and experimental results, can be challenging due to differences in data formats and structures.
Solution : Utilize data integration services and tools t hat standardize data formats and structures, enabling seamless merging of datasets. This may include employing middleware solutions or adopting APIs that facilitate data flow between systems. A unified data infrastructure enhances the comprehensiveness of analyses.
Resistance to Change
Researchers may be reluctant to adopt new analytics tools and methodologies due to familiarity with traditional practices or skepticism about the effectiveness of new approaches.
Solution : Provide comprehensive training and demonstrate the benefits of research analytics through case studies and pilot programs. Engaging researchers in hands-on workshops and showcasing success stories can help alleviate concerns and foster a culture of innovation.
Data Privacy Concerns
Ensuring compliance with data protection regulations, such as GDPR and HIPAA, is crucial in safeguarding participants’ rights and maintaining trust in the research process.
Solution : Establish clear data governance policies and practices, including data anonymization techniques, consent management systems, and regular compliance audits. Transparent communication about data handling practices can also enhance participant confidence.
Skill Gaps in Data Analysis
A lack of expertise in data analysis among researchers can hinder effective implementation and limit the potential of research analytics.
Solution : Invest in training programs to upskill existing staff and hire skilled analysts to bridge the knowledge gap. Collaborating with data experts or providing access to online courses can empower researchers to enhance their analytical skills and make data-driven decisions.
Wish to Maximize the Impact of Your Research with Expert Analytics?
Fill out The Form!
What is 1 + 1 =
Trends in Research Analytics
Research analytics is continuously evolving, shaped by advancements in technology and changing research needs. Several emerging trends are defining the landscape of research analytics:
AI-Driven Insights
- Leveraging artificial intelligence to enhance data analysis and predictive modeling allows researchers to gain deeper insights from complex datasets.
- With the support of AI integration services , you can identify patterns and correlations that may not be immediately visible. It helps in proactive decision-making and forecasting in research outcomes.
Open Science Initiatives
- Increasing emphasis on transparency and collaboration through shared data and methodologies, which facilitates the validation and replication of research findings.
- Initiatives such as preprint servers and open-access journals are becoming more prevalent, promoting the sharing of knowledge and fostering a collaborative research environment.
Automated Data Analysis
- Tools that automate data processing and analysis, significantly reducing manual efforts and minimizing human errors, which can skew research results.
- Automation allows for real-time data analysis, enabling researchers to respond quickly to emerging trends and insights without delay.
Interdisciplinary Research
- Combining insights from various fields such as biology, engineering, and social sciences to address complex research questions that require a multifaceted approach.
- This trend encourages cross-disciplinary collaboration, leading to innovative solutions that integrate different methodologies and perspectives, ultimately enhancing the overall quality of research.
Focus on Ethical Data Usage
- Prioritizing ethical considerations and compliance in data collection and analysis, ensuring that research adheres to standards of integrity and respect for participants’ rights.
- This trend includes implementing data governance frameworks, obtaining informed consent, and ensuring transparency in how data is used and reported, thereby fostering trust among research participants and stakeholders.
Elevate Your Research with Cutting-Edge Analytics and Latest Trends!
What is 2 + 2 =
How Can Matellio Help with Research Analytics?
Research analytics is essential for driving innovation, enhancing decision-making, and maximizing the impact of your research initiatives. Leveraging advanced analytics solutions can significantly boost your organization’s ability to extract meaningful insights from complex data and streamline research processes.
Choosing Matellio can be advantageous:
- Our team develops advanced analytics models tailored to your research needs. Whether you’re analyzing vast datasets, assessing research outcomes, or tracking progress.
- We implement predictive analytics to forecast research trends, identify emerging areas of interest, and assess potential risks. With this approach you to stay ahead of the curve, optimizing research strategies and resource allocation.
- Our expert guidance ensures the successful implementation and optimization of research analytics solutions. We work closely with your team to fully leverage data insights, enhance decision-making, improve research quality, and streamline workflows.
- We utilize cloud platforms to centralize access to research analytics tools and insights, facilitating better collaboration among your researchers. Our cloud integration services ensure that your research data is securely managed and easily accessible. It improves the efficiency and effectiveness of your data-driven research initiatives.
You can fill out the form and reach out for our expert guidance to explore how you can optimize your research initiatives with advanced analytics solutions.
FAQ’s
Q1. can research analytics integrate with existing research management systems.
Yes, research analytics solutions can be seamlessly integrated with existing research management systems, including platforms like LabKey, REDCap , and custom-built systems. We design solutions that ensure a smooth integration process, allowing for continuity in your research operations.
Q2. What are the costs associated with implementing research analytics?
Costs for implementing research analytics vary based on factors such as system complexity, data volume, and specific research requirements. We provide clear, detailed estimates tailored to your specific needs and budget.
Q3. What support and maintenance do you offer for research analytics solutions?
We offer comprehensive support, including 24/7 assistance , regular system updates, performance optimization, and proactive monitoring. Our goal is to ensure that your research analytics system remains effective, reliable, and up to date .
Q4. How is data migration handled during the implementation of research analytics?
Data migration is managed with a secure and structured approach, involving detailed planning and testing. We use backup and recovery solutions to ensure data integrity and minimize disruption during the transition.
Q5. How does Matellio ensure data security and compliance in research analytics solutions?
We implement robust security measures, including encryption, access controls, and regular audits, to ensure data protection and regulatory compliance. Our approach safeguards sensitive research data and maintains the integrity of your research analytics system.
Related articles
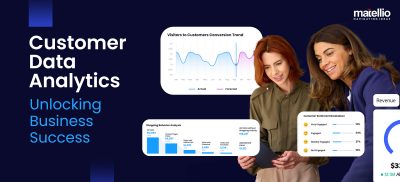
Customer Data Analytics: Unlocking Business Success
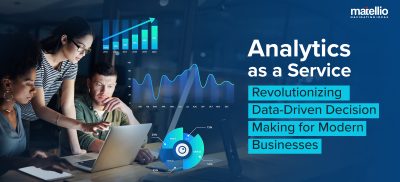
Analytics as a Service: Revolutionizing Data-Driven Decision Making for Modern Businesses
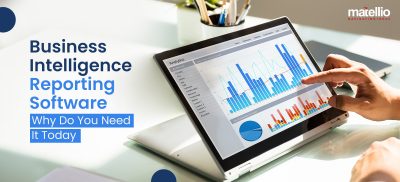
Business Intelligence Reporting Software – Why Do You Need It Today
Enquire now.
Facing Development Hurdles? Let’s Conquer Them Together. Our expert team is ready to tackle your challenges, from streamlining processes to scaling your tech.
Tactical Menu
Research, innovation & impact, research analytics.

The Research Analytics (RA) team in the Division of Research, Innovation & Impact provides research and sponsored projects reporting, analyses and strategic decision support for the MU community. Our work products can be categorized into:
- Routine reporting that is accurate, insightful and timely.
- Strategic decision support analyses for trends, goal setting, national benchmarking and targeted initiatives.
For each product, we strive to:
- Provide deliverables appropriate to the use case, customizing the format and level of detail to the request.
- Apply a professional “analyst” lens before sharing the deliverable so that you receive the most strategic support you need without needing to learn all aspects of the data.
Scope of work
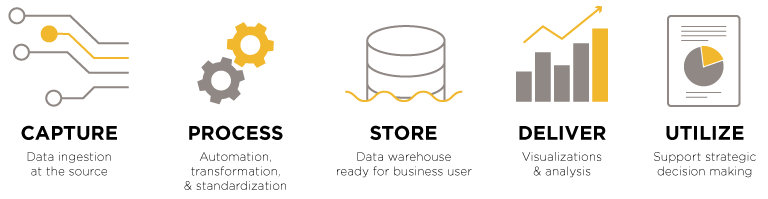
We use data from PeopleSoft grants, HR and financial systems to report on the proposal, award and expenditure (PAE) activity of our investigators. Sponsored projects are administrated through PeopleSoft grants and the PSRS is a key source of data for many of our reports. A sponsored project is scholarly activity conducted by MU that has 1) a principal investigator (PI) or program director (PD), 2) a budget and 3) a written project aim/scope of work.
In addition to sponsored project reporting, Research Analytics also provides research activity reporting. Not all research activity is sponsored; some is internally funded by departments and the division. All research activity is reported in the National Science Foundation HERD survey .
The Research Analytics team also works with investigators to understand sponsored data and identify how to update data if necessary. We work closely with the division’s Sponsored Programs Administration (SPA) for these activities.
Our team also collaborates with colleagues across the system to standardize reporting terminology, improve data quality and processes, create data warehouse reporting tables and set administrative data strategy for the division.
For questions related to only faculty (Academic Analytics, myVITA), student, HR or financial data, please visit other MU team pages for information. Research Analytics works with these teams to improve data quality and strategy; however, the team does not manage these data sets and does not report on them.
You may submit a request to [email protected] for many reasons, such as:
- Identifying experts on nanotechnology for a panel discussion and you would like to know who has funding in this area.
- Reviewing trends for activity with a specific funder to set baselines for goal setting.
- Finding eligible early career faculty that may be interested in applying to the NSF CAREER award.
- Receiving a summary of your own sponsored activity to see if the data was correctly entered before the promotion and tenure process.
- Obtaining a Power BI graphic that summarizes current MU funding from a specific sponsor or within a specific college, school or department.
MU RA manages a listserv for the higher education research analytics/sponsored data reporting community. This listserv has nearly 100 institutions participating and can be used to share job postings, gather insights on reporting best practices and to find like-minded colleagues. To subscribe to this email listserv please see these instructions . Members can interact with each other by sending listserv emails to: [email protected] .
If you are a vendor interested in joining the listserv, please understand that the listserv is not for advertising products and services. We welcome vendor participation in conversations on training, collaboration and tool usage. By requesting access, you are agreeing to these guidelines.
We are soliciting ideas for how to engage colleagues in research analytics and utilize this listserv. Please email us if you would like to host a webinar or have other ideas for engagement.

Program Evaluation
Data analytics.
- Psychometrics & Measurement
- Our Approach
- Areas Served
- Business & Corporations
- Higher Education
We are here to help you
Customized research & evaluation for precise insights.
Our analytics and data science research knowledge empowers your organization to make data-driven evaluation decisions with confidence.
Trusted by Top organizations

Examples of our impactful work

George W. Bush Institute School Leadership Initiative
Enhancing leadership in schools to drive student achievement through comprehensive training and support.

Understanding Determinants of Teen Pregnancy Prevention Programs
Analyzing factors that influence the effectiveness of teen pregnancy prevention efforts.

Determining the Impact of a Unique Public Health Initiative
Evaluate the outcomes and effectiveness of an innovative public health program on community well-being.

A Psychometric Approach to Accreditation
Applying psychometric methods to improve the reliability and validity of accreditation processes.
Over 20 Years of Research Analysis Consulting Experience
Wise words from our founder.
“Decreasing measurement error has been the focus of my entire career. It leads to more impactful decision making and more robust statistical modeling .”
Cindy M. Walker, PhD
Quantitative and Evaluative Research Methodologies

Our Experts
Tom schmitt.
Educational Statistics and Measurement
Sakine Gocer Sahin
Educational Measurement and Evaluation
James Walters
Mathematics, Statistics and Computer Science
Jacqueline Gosz

Focused on Results!
Our analytics and data science research knowledge empowers your organization to make data-driven decisions.
Schedule your consultation
Our team of experienced professionals is ready to help you achieve precise and actionable insights through customized research and evaluation services.
Fill out the form below to schedule your free initial consultation.

Psychometrics & Assessment

Let's keep connected
Fill in the form below to stay up-to-date with the latest news and, insights from RAC
- Skip to main content
- Skip to primary sidebar
- Skip to footer
- QuestionPro

- Solutions Industries Gaming Automotive Sports and events Education Government Travel & Hospitality Financial Services Healthcare Cannabis Technology Use Case AskWhy Communities Audience Contactless surveys Mobile LivePolls Member Experience GDPR Positive People Science 360 Feedback Surveys
- Resources Blog eBooks Survey Templates Case Studies Training Help center

Home Market Research
Data Analysis in Research: Types & Methods
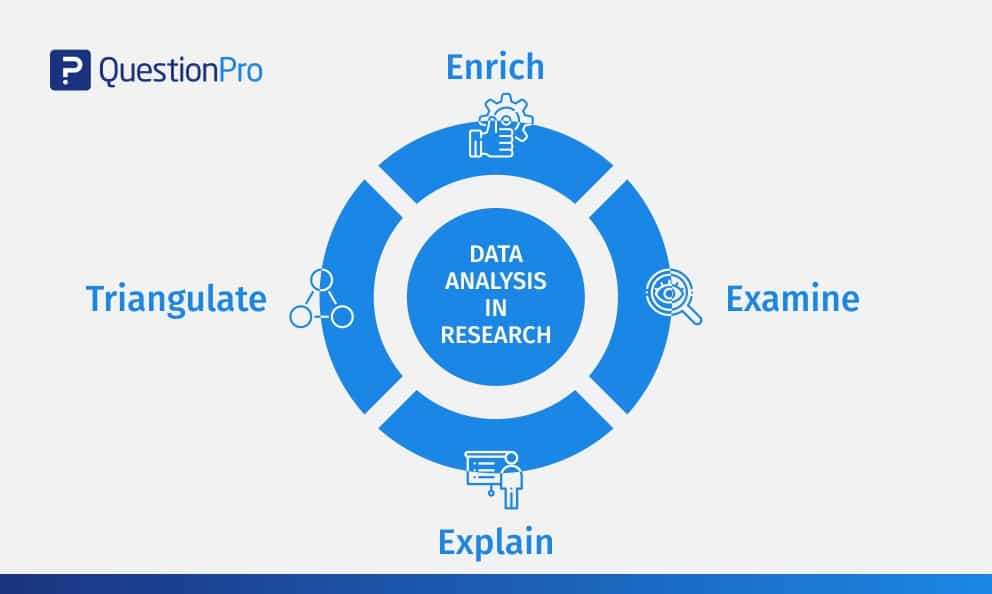
What is data analysis in research?
Definition of research in data analysis: According to LeCompte and Schensul, research data analysis is a process used by researchers to reduce data to a story and interpret it to derive insights. The data analysis process helps reduce a large chunk of data into smaller fragments, which makes sense.
Three essential things occur during the data analysis process — the first is data organization . Summarization and categorization together contribute to becoming the second known method used for data reduction. It helps find patterns and themes in the data for easy identification and linking. The third and last way is data analysis – researchers do it in both top-down and bottom-up fashion.
On the other hand, Marshall and Rossman describe data analysis as a messy, ambiguous, and time-consuming but creative and fascinating process through which a mass of collected data is brought to order, structure and meaning.
We can say that “the data analysis and data interpretation is a process representing the application of deductive and inductive logic to the research and data analysis.”
Why analyze data in research?
Researchers rely heavily on data as they have a story to tell or research problems to solve. It starts with a question, and data is nothing but an answer to that question. But, what if there is no question to ask? Well! It is possible to explore data even without a problem – we call it ‘Data Mining’, which often reveals some interesting patterns within the data that are worth exploring.
Irrelevant to the type of data researchers explore, their mission and audiences’ vision guide them to find the patterns to shape the story they want to tell. One of the essential things expected from researchers while analyzing data is to stay open and remain unbiased toward unexpected patterns, expressions, and results. Remember, sometimes, data analysis tells the most unforeseen yet exciting stories that were not expected when initiating data analysis. Therefore, rely on the data you have at hand and enjoy the journey of exploratory research.
Create a Free Account
Types of data in research
Every kind of data has a rare quality of describing things after assigning a specific value to it. For analysis, you need to organize these values, processed and presented in a given context, to make it useful. Data can be in different forms; here are the primary data types.
- Qualitative data: When the data presented has words and descriptions, then we call it qualitative data . Although you can observe this data, it is subjective and harder to analyze data in research, especially for comparison. Example: Quality data represents everything describing taste, experience, texture, or an opinion that is considered quality data. This type of data is usually collected through focus groups, personal qualitative interviews , qualitative observation or using open-ended questions in surveys.
- Quantitative data: Any data expressed in numbers of numerical figures are called quantitative data . This type of data can be distinguished into categories, grouped, measured, calculated, or ranked. Example: questions such as age, rank, cost, length, weight, scores, etc. everything comes under this type of data. You can present such data in graphical format, charts, or apply statistical analysis methods to this data. The (Outcomes Measurement Systems) OMS questionnaires in surveys are a significant source of collecting numeric data.
- Categorical data : It is data presented in groups. However, an item included in the categorical data cannot belong to more than one group. Example: A person responding to a survey by telling his living style, marital status, smoking habit, or drinking habit comes under the categorical data. A chi-square test is a standard method used to analyze this data.
Learn More : Examples of Qualitative Data in Education
Data analysis in qualitative research
Data analysis and qualitative data research work a little differently from the numerical data as the quality data is made up of words, descriptions, images, objects, and sometimes symbols. Getting insight from such complicated information is a complicated process. Hence it is typically used for exploratory research and data analysis .
Finding patterns in the qualitative data
Although there are several ways to find patterns in the textual information, a word-based method is the most relied and widely used global technique for research and data analysis. Notably, the data analysis process in qualitative research is manual. Here the researchers usually read the available data and find repetitive or commonly used words.
For example, while studying data collected from African countries to understand the most pressing issues people face, researchers might find “food” and “hunger” are the most commonly used words and will highlight them for further analysis.
The keyword context is another widely used word-based technique. In this method, the researcher tries to understand the concept by analyzing the context in which the participants use a particular keyword.
For example , researchers conducting research and data analysis for studying the concept of ‘diabetes’ amongst respondents might analyze the context of when and how the respondent has used or referred to the word ‘diabetes.’
The scrutiny-based technique is also one of the highly recommended text analysis methods used to identify a quality data pattern. Compare and contrast is the widely used method under this technique to differentiate how a specific text is similar or different from each other.
For example: To find out the “importance of resident doctor in a company,” the collected data is divided into people who think it is necessary to hire a resident doctor and those who think it is unnecessary. Compare and contrast is the best method that can be used to analyze the polls having single-answer questions types .
Metaphors can be used to reduce the data pile and find patterns in it so that it becomes easier to connect data with theory.
Variable Partitioning is another technique used to split variables so that researchers can find more coherent descriptions and explanations from the enormous data.
Methods used for data analysis in qualitative research
There are several techniques to analyze the data in qualitative research, but here are some commonly used methods,
- Content Analysis: It is widely accepted and the most frequently employed technique for data analysis in research methodology. It can be used to analyze the documented information from text, images, and sometimes from the physical items. It depends on the research questions to predict when and where to use this method.
- Narrative Analysis: This method is used to analyze content gathered from various sources such as personal interviews, field observation, and surveys . The majority of times, stories, or opinions shared by people are focused on finding answers to the research questions.
- Discourse Analysis: Similar to narrative analysis, discourse analysis is used to analyze the interactions with people. Nevertheless, this particular method considers the social context under which or within which the communication between the researcher and respondent takes place. In addition to that, discourse analysis also focuses on the lifestyle and day-to-day environment while deriving any conclusion.
- Grounded Theory: When you want to explain why a particular phenomenon happened, then using grounded theory for analyzing quality data is the best resort. Grounded theory is applied to study data about the host of similar cases occurring in different settings. When researchers are using this method, they might alter explanations or produce new ones until they arrive at some conclusion.
Choosing the right software can be tough. Whether you’re a researcher, business leader, or marketer, check out the top 10 qualitative data analysis software for analyzing qualitative data.
Data analysis in quantitative research
Preparing data for analysis.
The first stage in research and data analysis is to make it for the analysis so that the nominal data can be converted into something meaningful. Data preparation consists of the below phases.
Phase I: Data Validation
Data validation is done to understand if the collected data sample is per the pre-set standards, or it is a biased data sample again divided into four different stages
- Fraud: To ensure an actual human being records each response to the survey or the questionnaire
- Screening: To make sure each participant or respondent is selected or chosen in compliance with the research criteria
- Procedure: To ensure ethical standards were maintained while collecting the data sample
- Completeness: To ensure that the respondent has answered all the questions in an online survey. Else, the interviewer had asked all the questions devised in the questionnaire.
Phase II: Data Editing
More often, an extensive research data sample comes loaded with errors. Respondents sometimes fill in some fields incorrectly or sometimes skip them accidentally. Data editing is a process wherein the researchers have to confirm that the provided data is free of such errors. They need to conduct necessary checks and outlier checks to edit the raw edit and make it ready for analysis.
Phase III: Data Coding
Out of all three, this is the most critical phase of data preparation associated with grouping and assigning values to the survey responses . If a survey is completed with a 1000 sample size, the researcher will create an age bracket to distinguish the respondents based on their age. Thus, it becomes easier to analyze small data buckets rather than deal with the massive data pile.
LEARN ABOUT: Steps in Qualitative Research
Methods used for data analysis in quantitative research
After the data is prepared for analysis, researchers are open to using different research and data analysis methods to derive meaningful insights. For sure, statistical analysis plans are the most favored to analyze numerical data. In statistical analysis, distinguishing between categorical data and numerical data is essential, as categorical data involves distinct categories or labels, while numerical data consists of measurable quantities. The method is again classified into two groups. First, ‘Descriptive Statistics’ used to describe data. Second, ‘Inferential statistics’ that helps in comparing the data .
Descriptive statistics
This method is used to describe the basic features of versatile types of data in research. It presents the data in such a meaningful way that pattern in the data starts making sense. Nevertheless, the descriptive analysis does not go beyond making conclusions. The conclusions are again based on the hypothesis researchers have formulated so far. Here are a few major types of descriptive analysis methods.
Measures of Frequency
- Count, Percent, Frequency
- It is used to denote home often a particular event occurs.
- Researchers use it when they want to showcase how often a response is given.
Measures of Central Tendency
- Mean, Median, Mode
- The method is widely used to demonstrate distribution by various points.
- Researchers use this method when they want to showcase the most commonly or averagely indicated response.

Measures of Dispersion or Variation
- Range, Variance, Standard deviation
- Here the field equals high/low points.
- Variance standard deviation = difference between the observed score and mean
- It is used to identify the spread of scores by stating intervals.
- Researchers use this method to showcase data spread out. It helps them identify the depth until which the data is spread out that it directly affects the mean.
Measures of Position
- Percentile ranks, Quartile ranks
- It relies on standardized scores helping researchers to identify the relationship between different scores.
- It is often used when researchers want to compare scores with the average count.
For quantitative research use of descriptive analysis often give absolute numbers, but the in-depth analysis is never sufficient to demonstrate the rationale behind those numbers. Nevertheless, it is necessary to think of the best method for research and data analysis suiting your survey questionnaire and what story researchers want to tell. For example, the mean is the best way to demonstrate the students’ average scores in schools. It is better to rely on the descriptive statistics when the researchers intend to keep the research or outcome limited to the provided sample without generalizing it. For example, when you want to compare average voting done in two different cities, differential statistics are enough.
Descriptive analysis is also called a ‘univariate analysis’ since it is commonly used to analyze a single variable.
Inferential statistics
Inferential statistics are used to make predictions about a larger population after research and data analysis of the representing population’s collected sample. For example, you can ask some odd 100 audiences at a movie theater if they like the movie they are watching. Researchers then use inferential statistics on the collected sample to reason that about 80-90% of people like the movie.
Here are two significant areas of inferential statistics.
- Estimating parameters: It takes statistics from the sample research data and demonstrates something about the population parameter.
- Hypothesis test: I t’s about sampling research data to answer the survey research questions. For example, researchers might be interested to understand if the new shade of lipstick recently launched is good or not, or if the multivitamin capsules help children to perform better at games.
These are sophisticated analysis methods used to showcase the relationship between different variables instead of describing a single variable. It is often used when researchers want something beyond absolute numbers to understand the relationship between variables.
Here are some of the commonly used methods for data analysis in research.
- Correlation: When researchers are not conducting experimental research or quasi-experimental research wherein the researchers are interested to understand the relationship between two or more variables, they opt for correlational research methods.
- Cross-tabulation: Also called contingency tables, cross-tabulation is used to analyze the relationship between multiple variables. Suppose provided data has age and gender categories presented in rows and columns. A two-dimensional cross-tabulation helps for seamless data analysis and research by showing the number of males and females in each age category.
- Regression analysis: For understanding the strong relationship between two variables, researchers do not look beyond the primary and commonly used regression analysis method, which is also a type of predictive analysis used. In this method, you have an essential factor called the dependent variable. You also have multiple independent variables in regression analysis. You undertake efforts to find out the impact of independent variables on the dependent variable. The values of both independent and dependent variables are assumed as being ascertained in an error-free random manner.
- Frequency tables: The statistical procedure is used for testing the degree to which two or more vary or differ in an experiment. A considerable degree of variation means research findings were significant. In many contexts, ANOVA testing and variance analysis are similar.
- Analysis of variance: The statistical procedure is used for testing the degree to which two or more vary or differ in an experiment. A considerable degree of variation means research findings were significant. In many contexts, ANOVA testing and variance analysis are similar.
Considerations in research data analysis
- Researchers must have the necessary research skills to analyze and manipulation the data , Getting trained to demonstrate a high standard of research practice. Ideally, researchers must possess more than a basic understanding of the rationale of selecting one statistical method over the other to obtain better data insights.
- Usually, research and data analytics projects differ by scientific discipline; therefore, getting statistical advice at the beginning of analysis helps design a survey questionnaire, select data collection methods , and choose samples.
LEARN ABOUT: Best Data Collection Tools
- The primary aim of data research and analysis is to derive ultimate insights that are unbiased. Any mistake in or keeping a biased mind to collect data, selecting an analysis method, or choosing audience sample il to draw a biased inference.
- Irrelevant to the sophistication used in research data and analysis is enough to rectify the poorly defined objective outcome measurements. It does not matter if the design is at fault or intentions are not clear, but lack of clarity might mislead readers, so avoid the practice.
- The motive behind data analysis in research is to present accurate and reliable data. As far as possible, avoid statistical errors, and find a way to deal with everyday challenges like outliers, missing data, data altering, data mining , or developing graphical representation.
LEARN MORE: Descriptive Research vs Correlational Research The sheer amount of data generated daily is frightening. Especially when data analysis has taken center stage. in 2018. In last year, the total data supply amounted to 2.8 trillion gigabytes. Hence, it is clear that the enterprises willing to survive in the hypercompetitive world must possess an excellent capability to analyze complex research data, derive actionable insights, and adapt to the new market needs.
LEARN ABOUT: Average Order Value
QuestionPro is an online survey platform that empowers organizations in data analysis and research and provides them a medium to collect data by creating appealing surveys.
MORE LIKE THIS
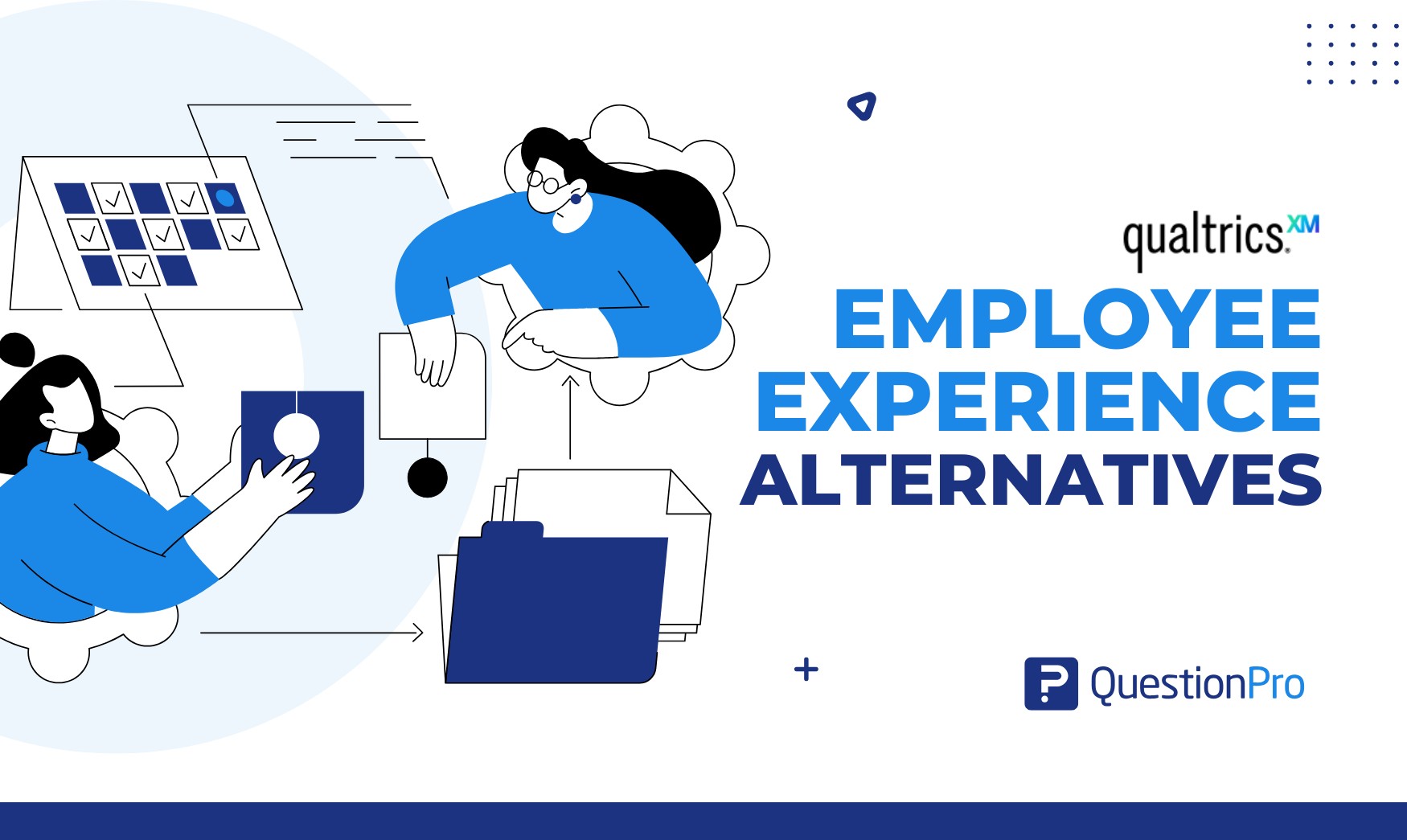
Qualtrics Employee Experience Alternatives: The 6 Best in 2024
Nov 19, 2024
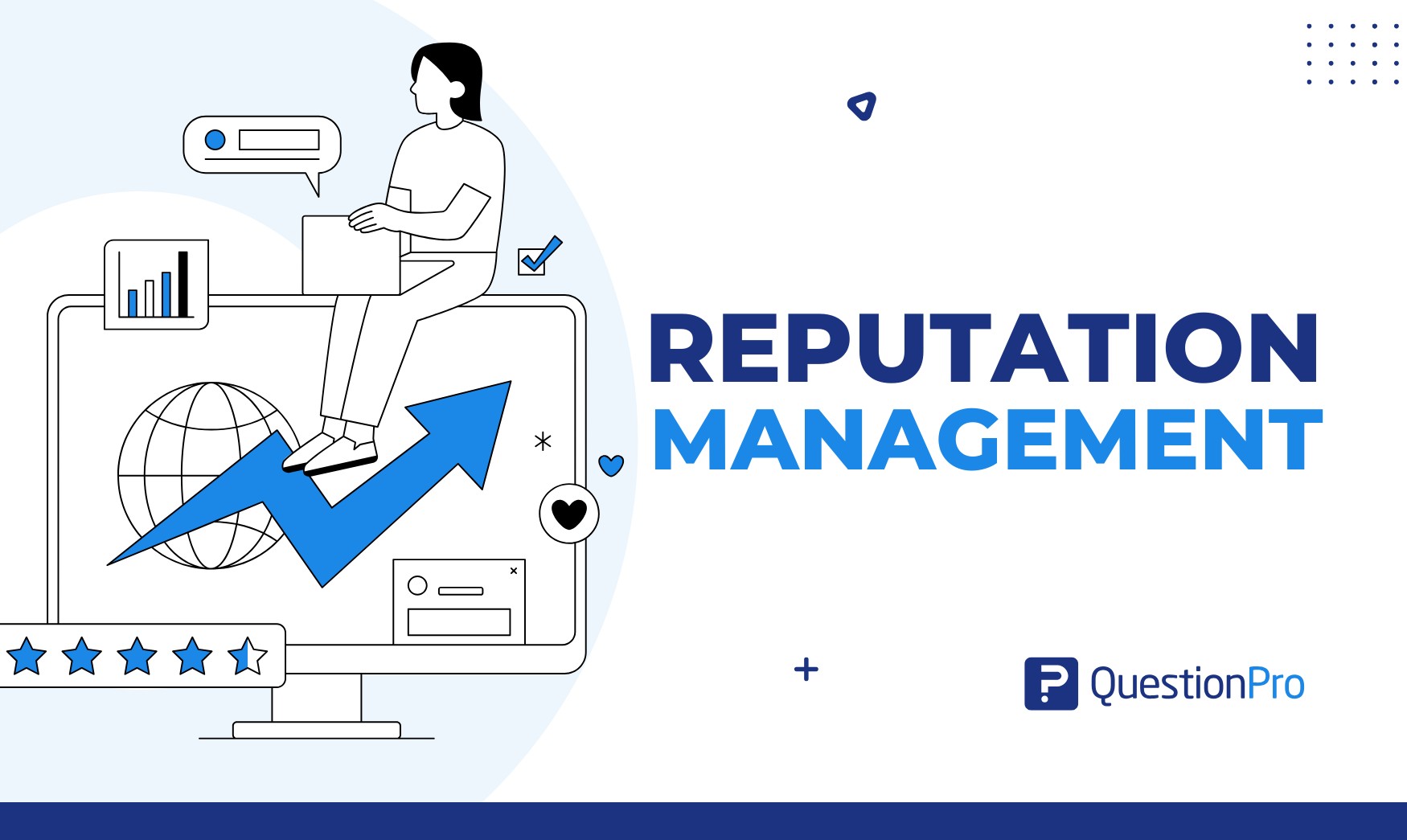
Reputation Management: How to Protect Your Brand Reputation?
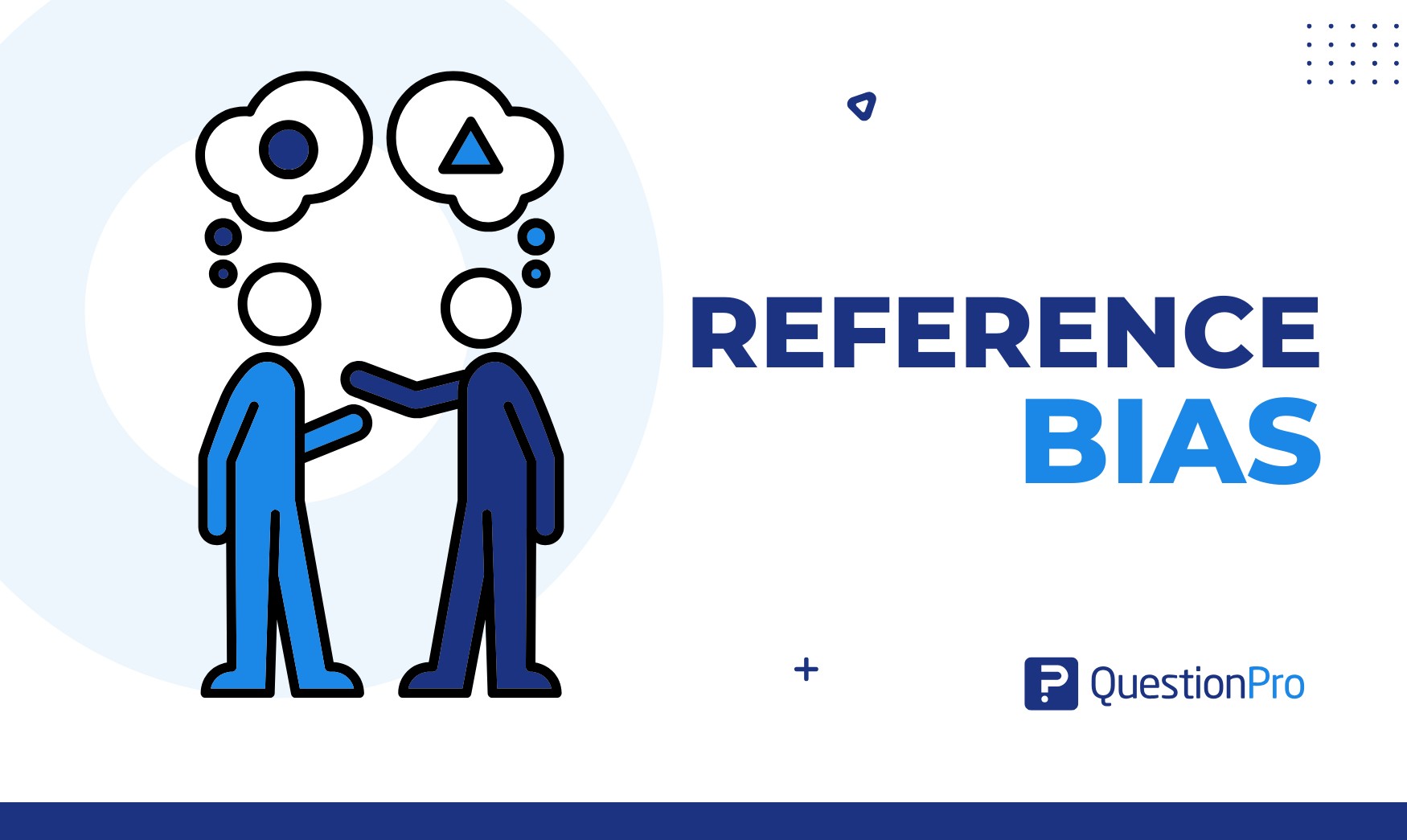
Reference Bias: Identifying and Reducing in Surveys and Research
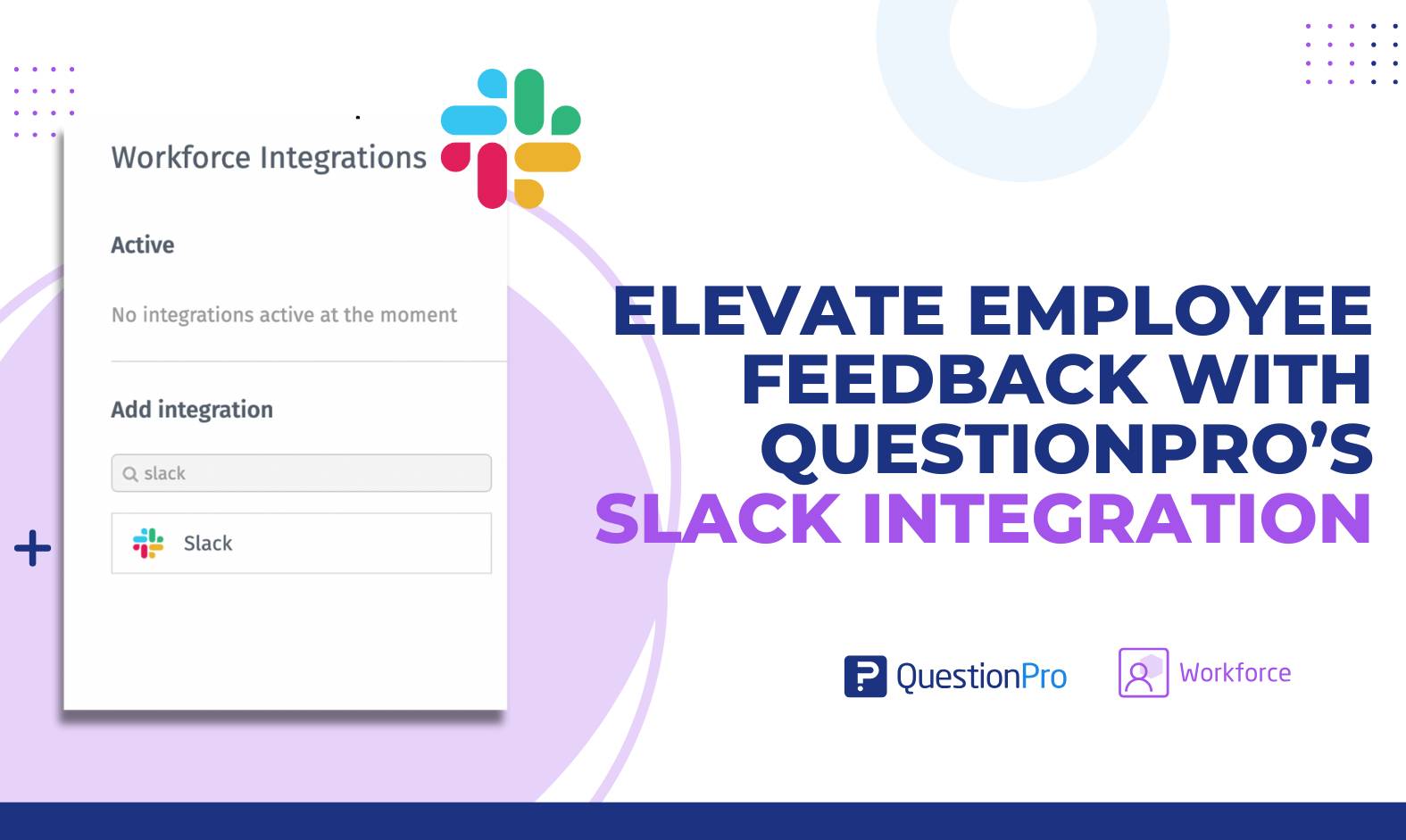
Maximize Employee Feedback with QuestionPro Workforce’s Slack Integration
Nov 6, 2024
Other categories
- Academic Research
- Artificial Intelligence
- Assessments
- Brand Awareness
- Case Studies
- Communities
- Consumer Insights
- Customer effort score
- Customer Engagement
- Customer Experience
- Customer Loyalty
- Customer Research
- Customer Satisfaction
- Employee Benefits
- Employee Engagement
- Employee Retention
- Friday Five
- General Data Protection Regulation
- Insights Hub
- Life@QuestionPro
- Market Research
- Mobile diaries
- Mobile Surveys
- New Features
- Online Communities
- Question Types
- Questionnaire
- QuestionPro Products
- Release Notes
- Research Tools and Apps
- Revenue at Risk
- Survey Templates
- Training Tips
- Tuesday CX Thoughts (TCXT)
- Uncategorized
- What’s Coming Up
- Workforce Intelligence
- Privacy Policy

Home » Data Analysis – Process, Methods and Types
Data Analysis – Process, Methods and Types
Table of Contents
Data analysis is the systematic process of inspecting, cleaning, transforming, and modeling data to uncover meaningful insights, support decision-making, and solve specific problems. In today’s data-driven world, data analysis is crucial for businesses, researchers, and policymakers to interpret trends, predict outcomes, and make informed decisions. This article delves into the data analysis process, commonly used methods, and the different types of data analysis.

Data Analysis
Data analysis involves the application of statistical, mathematical, and computational techniques to make sense of raw data. It transforms unorganized data into actionable information, often through visualizations, statistical summaries, or predictive models.
For example, analyzing sales data over time can help a retailer understand seasonal trends and forecast future demand.
Importance of Data Analysis
- Informed Decision-Making: Helps stakeholders make evidence-based choices.
- Problem Solving: Identifies patterns, relationships, and anomalies in data.
- Efficiency Improvement: Optimizes processes and operations through insights.
- Strategic Planning: Assists in setting realistic goals and forecasting outcomes.
Data Analysis Process
The process of data analysis typically follows a structured approach to ensure accuracy and reliability.
1. Define Objectives
Clearly articulate the research question or business problem you aim to address.
- Example: A company wants to analyze customer satisfaction to improve its services.
2. Data Collection
Gather relevant data from various sources, such as surveys, databases, or APIs.
- Example: Collect customer feedback through online surveys and customer service logs.
3. Data Cleaning
Prepare the data for analysis by removing errors, duplicates, and inconsistencies.
- Example: Handle missing values, correct typos, and standardize formats.
4. Data Exploration
Perform exploratory data analysis (EDA) to understand data patterns, distributions, and relationships.
- Example: Use summary statistics and visualizations like histograms or scatter plots.
5. Data Transformation
Transform raw data into a usable format by scaling, encoding, or aggregating.
- Example: Convert categorical data into numerical values for machine learning algorithms.
6. Analysis and Interpretation
Apply appropriate methods or models to analyze the data and extract insights.
- Example: Use regression analysis to predict customer churn rates.
7. Reporting and Visualization
Present findings in a clear and actionable format using dashboards, charts, or reports.
- Example: Create a dashboard summarizing customer satisfaction scores by region.
8. Decision-Making and Implementation
Use the insights to make recommendations or implement strategies.
- Example: Launch targeted marketing campaigns based on customer preferences.
Methods of Data Analysis
1. statistical methods.
- Descriptive Statistics: Summarizes data using measures like mean, median, and standard deviation.
- Inferential Statistics: Draws conclusions or predictions from sample data using techniques like hypothesis testing or confidence intervals.
2. Data Mining
Data mining involves discovering patterns, correlations, and anomalies in large datasets.
- Example: Identifying purchasing patterns in retail through association rules.
3. Machine Learning
Applies algorithms to build predictive models and automate decision-making.
- Example: Using supervised learning to classify email spam.
4. Text Analysis
Analyzes textual data to extract insights, often used in sentiment analysis or topic modeling.
- Example: Analyzing customer reviews to understand product sentiment.
5. Time-Series Analysis
Focuses on analyzing data points collected over time to identify trends and patterns.
- Example: Forecasting stock prices based on historical data.
6. Data Visualization
Transforms data into visual representations like charts, graphs, and heatmaps to make findings comprehensible.
- Example: Using bar charts to compare monthly sales performance.
7. Predictive Analytics
Uses statistical models and machine learning to forecast future outcomes based on historical data.
- Example: Predicting the likelihood of equipment failure in a manufacturing plant.
8. Diagnostic Analysis
Focuses on identifying causes of observed patterns or trends in data.
- Example: Investigating why sales dropped in a particular quarter.
Types of Data Analysis
1. descriptive analysis.
- Purpose: Summarizes raw data to provide insights into past trends and performance.
- Example: Analyzing average customer spending per month.
2. Exploratory Analysis
- Purpose: Identifies patterns, relationships, or hypotheses for further study.
- Example: Exploring correlations between advertising spend and sales.
3. Inferential Analysis
- Purpose: Draws conclusions or makes predictions about a population based on sample data.
- Example: Estimating national voter preferences using survey data.
4. Diagnostic Analysis
- Purpose: Examines the reasons behind observed outcomes or trends.
- Example: Investigating why website traffic decreased after a redesign.
5. Predictive Analysis
- Purpose: Forecasts future outcomes based on historical data.
- Example: Predicting customer churn using machine learning algorithms.
6. Prescriptive Analysis
- Purpose: Recommends actions based on data insights and predictive models.
- Example: Suggesting the best marketing channels to maximize ROI.
Tools for Data Analysis
1. programming languages.
- Python: Popular for data manipulation, analysis, and machine learning (e.g., Pandas, NumPy, Scikit-learn).
- R: Ideal for statistical computing and visualization.
2. Data Visualization Tools
- Tableau: Creates interactive dashboards and visualizations.
- Power BI: Microsoft’s tool for business intelligence and reporting.
3. Statistical Software
- SPSS: Used for statistical analysis in social sciences.
- SAS: Advanced analytics, data management, and predictive modeling tool.
4. Big Data Platforms
- Hadoop: Framework for processing large-scale datasets.
- Apache Spark: Fast data processing engine for big data analytics.
5. Spreadsheet Tools
- Microsoft Excel: Widely used for basic data analysis and visualization.
- Google Sheets: Collaborative online spreadsheet tool.
Challenges in Data Analysis
- Data Quality Issues: Missing, inconsistent, or inaccurate data can compromise results.
- Scalability: Analyzing large datasets requires advanced tools and computing power.
- Bias in Data: Skewed datasets can lead to misleading conclusions.
- Complexity: Choosing the appropriate analysis methods and models can be challenging.
Applications of Data Analysis
- Business: Improving customer experience through sales and marketing analytics.
- Healthcare: Analyzing patient data to improve treatment outcomes.
- Education: Evaluating student performance and designing effective teaching strategies.
- Finance: Detecting fraudulent transactions using predictive models.
- Social Science: Understanding societal trends through demographic analysis.
Data analysis is an essential process for transforming raw data into actionable insights. By understanding the process, methods, and types of data analysis, researchers and professionals can effectively tackle complex problems, uncover trends, and make data-driven decisions. With advancements in tools and technology, the scope and impact of data analysis continue to expand, shaping the future of industries and research.
- McKinney, W. (2017). Python for Data Analysis: Data Wrangling with Pandas, NumPy, and IPython . O’Reilly Media.
- Han, J., Pei, J., & Kamber, M. (2011). Data Mining: Concepts and Techniques . Morgan Kaufmann.
- Provost, F., & Fawcett, T. (2013). Data Science for Business: What You Need to Know About Data Mining and Data-Analytic Thinking . O’Reilly Media.
- Montgomery, D. C., & Runger, G. C. (2018). Applied Statistics and Probability for Engineers . Wiley.
- Tableau Public (2023). Creating Data Visualizations and Dashboards . Retrieved from https://www.tableau.com.
About the author
Muhammad Hassan
Researcher, Academic Writer, Web developer
You may also like

Research Techniques – Methods, Types and Examples

What is Research Topic – Ideas and Examples

Research Gap – Types, Examples and How to...

Research Results Section – Writing Guide and...

Symmetric Histogram – Examples and Making Guide

Research Objectives – Types, Examples and...
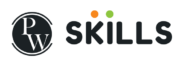
Data Analysis Techniques in Research – Methods, Tools & Examples
Varun Saharawat is a seasoned professional in the fields of SEO and content writing. With a profound knowledge of the intricate aspects of these disciplines, Varun has established himself as a valuable asset in the world of digital marketing and online content creation.
Data analysis techniques in research are essential because they allow researchers to derive meaningful insights from data sets to support their hypotheses or research objectives.
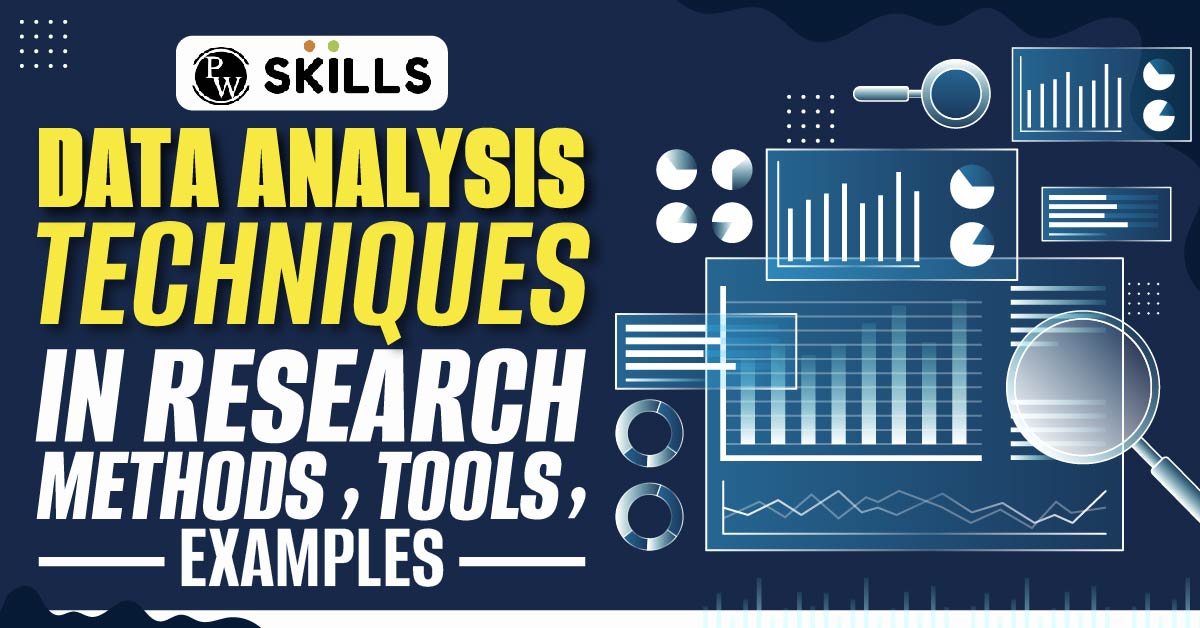
Data Analysis Techniques in Research : While various groups, institutions, and professionals may have diverse approaches to data analysis, a universal definition captures its essence. Data analysis involves refining, transforming, and interpreting raw data to derive actionable insights that guide informed decision-making for businesses.
A straightforward illustration of data analysis emerges when we make everyday decisions, basing our choices on past experiences or predictions of potential outcomes.
If you want to learn more about this topic and acquire valuable skills that will set you apart in today’s data-driven world, we highly recommend enrolling in the Data Analytics Course by Physics Wallah . And as a special offer for our readers, use the coupon code “READER” to get a discount on this course.
Table of Contents
What is Data Analysis?
Data analysis is the systematic process of inspecting, cleaning, transforming, and interpreting data with the objective of discovering valuable insights and drawing meaningful conclusions. This process involves several steps:
- Inspecting : Initial examination of data to understand its structure, quality, and completeness.
- Cleaning : Removing errors, inconsistencies, or irrelevant information to ensure accurate analysis.
- Transforming : Converting data into a format suitable for analysis, such as normalization or aggregation.
- Interpreting : Analyzing the transformed data to identify patterns, trends, and relationships.
Types of Data Analysis Techniques in Research
Data analysis techniques in research are categorized into qualitative and quantitative methods, each with its specific approaches and tools. These techniques are instrumental in extracting meaningful insights, patterns, and relationships from data to support informed decision-making, validate hypotheses, and derive actionable recommendations. Below is an in-depth exploration of the various types of data analysis techniques commonly employed in research:
1) Qualitative Analysis:
Definition: Qualitative analysis focuses on understanding non-numerical data, such as opinions, concepts, or experiences, to derive insights into human behavior, attitudes, and perceptions.
- Content Analysis: Examines textual data, such as interview transcripts, articles, or open-ended survey responses, to identify themes, patterns, or trends.
- Narrative Analysis: Analyzes personal stories or narratives to understand individuals’ experiences, emotions, or perspectives.
- Ethnographic Studies: Involves observing and analyzing cultural practices, behaviors, and norms within specific communities or settings.
2) Quantitative Analysis:
Quantitative analysis emphasizes numerical data and employs statistical methods to explore relationships, patterns, and trends. It encompasses several approaches:
Descriptive Analysis:
- Frequency Distribution: Represents the number of occurrences of distinct values within a dataset.
- Central Tendency: Measures such as mean, median, and mode provide insights into the central values of a dataset.
- Dispersion: Techniques like variance and standard deviation indicate the spread or variability of data.
Diagnostic Analysis:
- Regression Analysis: Assesses the relationship between dependent and independent variables, enabling prediction or understanding causality.
- ANOVA (Analysis of Variance): Examines differences between groups to identify significant variations or effects.
Predictive Analysis:
- Time Series Forecasting: Uses historical data points to predict future trends or outcomes.
- Machine Learning Algorithms: Techniques like decision trees, random forests, and neural networks predict outcomes based on patterns in data.
Prescriptive Analysis:
- Optimization Models: Utilizes linear programming, integer programming, or other optimization techniques to identify the best solutions or strategies.
- Simulation: Mimics real-world scenarios to evaluate various strategies or decisions and determine optimal outcomes.
Specific Techniques:
- Monte Carlo Simulation: Models probabilistic outcomes to assess risk and uncertainty.
- Factor Analysis: Reduces the dimensionality of data by identifying underlying factors or components.
- Cohort Analysis: Studies specific groups or cohorts over time to understand trends, behaviors, or patterns within these groups.
- Cluster Analysis : Classifies objects or individuals into homogeneous groups or clusters based on similarities or attributes.
- Sentiment Analysis: Uses natural language processing and machine learning techniques to determine sentiment, emotions, or opinions from textual data.
Also Read: AI and Predictive Analytics: Examples, Tools, Uses, Ai Vs Predictive Analytics
Data Analysis Techniques in Research Examples
To provide a clearer understanding of how data analysis techniques are applied in research, let’s consider a hypothetical research study focused on evaluating the impact of online learning platforms on students’ academic performance.
Research Objective:
Determine if students using online learning platforms achieve higher academic performance compared to those relying solely on traditional classroom instruction.
Data Collection:
- Quantitative Data: Academic scores (grades) of students using online platforms and those using traditional classroom methods.
- Qualitative Data: Feedback from students regarding their learning experiences, challenges faced, and preferences.
Data Analysis Techniques Applied:
1) Descriptive Analysis:
- Calculate the mean, median, and mode of academic scores for both groups.
- Create frequency distributions to represent the distribution of grades in each group.
2) Diagnostic Analysis:
- Conduct an Analysis of Variance (ANOVA) to determine if there’s a statistically significant difference in academic scores between the two groups.
- Perform Regression Analysis to assess the relationship between the time spent on online platforms and academic performance.
3) Predictive Analysis:
- Utilize Time Series Forecasting to predict future academic performance trends based on historical data.
- Implement Machine Learning algorithms to develop a predictive model that identifies factors contributing to academic success on online platforms.
4) Prescriptive Analysis:
- Apply Optimization Models to identify the optimal combination of online learning resources (e.g., video lectures, interactive quizzes) that maximize academic performance.
- Use Simulation Techniques to evaluate different scenarios, such as varying student engagement levels with online resources, to determine the most effective strategies for improving learning outcomes.
5) Specific Techniques:
- Conduct Factor Analysis on qualitative feedback to identify common themes or factors influencing students’ perceptions and experiences with online learning.
- Perform Cluster Analysis to segment students based on their engagement levels, preferences, or academic outcomes, enabling targeted interventions or personalized learning strategies.
- Apply Sentiment Analysis on textual feedback to categorize students’ sentiments as positive, negative, or neutral regarding online learning experiences.
By applying a combination of qualitative and quantitative data analysis techniques, this research example aims to provide comprehensive insights into the effectiveness of online learning platforms.
Also Read: Learning Path to Become a Data Analyst in 2024
Data Analysis Techniques in Quantitative Research
Quantitative research involves collecting numerical data to examine relationships, test hypotheses, and make predictions. Various data analysis techniques are employed to interpret and draw conclusions from quantitative data. Here are some key data analysis techniques commonly used in quantitative research:
1) Descriptive Statistics:
- Description: Descriptive statistics are used to summarize and describe the main aspects of a dataset, such as central tendency (mean, median, mode), variability (range, variance, standard deviation), and distribution (skewness, kurtosis).
- Applications: Summarizing data, identifying patterns, and providing initial insights into the dataset.
2) Inferential Statistics:
- Description: Inferential statistics involve making predictions or inferences about a population based on a sample of data. This technique includes hypothesis testing, confidence intervals, t-tests, chi-square tests, analysis of variance (ANOVA), regression analysis, and correlation analysis.
- Applications: Testing hypotheses, making predictions, and generalizing findings from a sample to a larger population.
3) Regression Analysis:
- Description: Regression analysis is a statistical technique used to model and examine the relationship between a dependent variable and one or more independent variables. Linear regression, multiple regression, logistic regression, and nonlinear regression are common types of regression analysis .
- Applications: Predicting outcomes, identifying relationships between variables, and understanding the impact of independent variables on the dependent variable.
4) Correlation Analysis:
- Description: Correlation analysis is used to measure and assess the strength and direction of the relationship between two or more variables. The Pearson correlation coefficient, Spearman rank correlation coefficient, and Kendall’s tau are commonly used measures of correlation.
- Applications: Identifying associations between variables and assessing the degree and nature of the relationship.
5) Factor Analysis:
- Description: Factor analysis is a multivariate statistical technique used to identify and analyze underlying relationships or factors among a set of observed variables. It helps in reducing the dimensionality of data and identifying latent variables or constructs.
- Applications: Identifying underlying factors or constructs, simplifying data structures, and understanding the underlying relationships among variables.
6) Time Series Analysis:
- Description: Time series analysis involves analyzing data collected or recorded over a specific period at regular intervals to identify patterns, trends, and seasonality. Techniques such as moving averages, exponential smoothing, autoregressive integrated moving average (ARIMA), and Fourier analysis are used.
- Applications: Forecasting future trends, analyzing seasonal patterns, and understanding time-dependent relationships in data.
7) ANOVA (Analysis of Variance):
- Description: Analysis of variance (ANOVA) is a statistical technique used to analyze and compare the means of two or more groups or treatments to determine if they are statistically different from each other. One-way ANOVA, two-way ANOVA, and MANOVA (Multivariate Analysis of Variance) are common types of ANOVA.
- Applications: Comparing group means, testing hypotheses, and determining the effects of categorical independent variables on a continuous dependent variable.
8) Chi-Square Tests:
- Description: Chi-square tests are non-parametric statistical tests used to assess the association between categorical variables in a contingency table. The Chi-square test of independence, goodness-of-fit test, and test of homogeneity are common chi-square tests.
- Applications: Testing relationships between categorical variables, assessing goodness-of-fit, and evaluating independence.
These quantitative data analysis techniques provide researchers with valuable tools and methods to analyze, interpret, and derive meaningful insights from numerical data. The selection of a specific technique often depends on the research objectives, the nature of the data, and the underlying assumptions of the statistical methods being used.
Also Read: Analysis vs. Analytics: How Are They Different?
Data Analysis Methods
Data analysis methods refer to the techniques and procedures used to analyze, interpret, and draw conclusions from data. These methods are essential for transforming raw data into meaningful insights, facilitating decision-making processes, and driving strategies across various fields. Here are some common data analysis methods:
- Description: Descriptive statistics summarize and organize data to provide a clear and concise overview of the dataset. Measures such as mean, median, mode, range, variance, and standard deviation are commonly used.
- Description: Inferential statistics involve making predictions or inferences about a population based on a sample of data. Techniques such as hypothesis testing, confidence intervals, and regression analysis are used.
3) Exploratory Data Analysis (EDA):
- Description: EDA techniques involve visually exploring and analyzing data to discover patterns, relationships, anomalies, and insights. Methods such as scatter plots, histograms, box plots, and correlation matrices are utilized.
- Applications: Identifying trends, patterns, outliers, and relationships within the dataset.
4) Predictive Analytics:
- Description: Predictive analytics use statistical algorithms and machine learning techniques to analyze historical data and make predictions about future events or outcomes. Techniques such as regression analysis, time series forecasting, and machine learning algorithms (e.g., decision trees, random forests, neural networks) are employed.
- Applications: Forecasting future trends, predicting outcomes, and identifying potential risks or opportunities.
5) Prescriptive Analytics:
- Description: Prescriptive analytics involve analyzing data to recommend actions or strategies that optimize specific objectives or outcomes. Optimization techniques, simulation models, and decision-making algorithms are utilized.
- Applications: Recommending optimal strategies, decision-making support, and resource allocation.
6) Qualitative Data Analysis:
- Description: Qualitative data analysis involves analyzing non-numerical data, such as text, images, videos, or audio, to identify themes, patterns, and insights. Methods such as content analysis, thematic analysis, and narrative analysis are used.
- Applications: Understanding human behavior, attitudes, perceptions, and experiences.
7) Big Data Analytics:
- Description: Big data analytics methods are designed to analyze large volumes of structured and unstructured data to extract valuable insights. Technologies such as Hadoop, Spark, and NoSQL databases are used to process and analyze big data.
- Applications: Analyzing large datasets, identifying trends, patterns, and insights from big data sources.
8) Text Analytics:
- Description: Text analytics methods involve analyzing textual data, such as customer reviews, social media posts, emails, and documents, to extract meaningful information and insights. Techniques such as sentiment analysis, text mining, and natural language processing (NLP) are used.
- Applications: Analyzing customer feedback, monitoring brand reputation, and extracting insights from textual data sources.
These data analysis methods are instrumental in transforming data into actionable insights, informing decision-making processes, and driving organizational success across various sectors, including business, healthcare, finance, marketing, and research. The selection of a specific method often depends on the nature of the data, the research objectives, and the analytical requirements of the project or organization.
Also Read: Quantitative Data Analysis: Types, Analysis & Examples
Data Analysis Tools
Data analysis tools are essential instruments that facilitate the process of examining, cleaning, transforming, and modeling data to uncover useful information, make informed decisions, and drive strategies. Here are some prominent data analysis tools widely used across various industries:
1) Microsoft Excel:
- Description: A spreadsheet software that offers basic to advanced data analysis features, including pivot tables, data visualization tools, and statistical functions.
- Applications: Data cleaning, basic statistical analysis, visualization, and reporting.
2) R Programming Language :
- Description: An open-source programming language specifically designed for statistical computing and data visualization.
- Applications: Advanced statistical analysis, data manipulation, visualization, and machine learning.
3) Python (with Libraries like Pandas, NumPy, Matplotlib, and Seaborn):
- Description: A versatile programming language with libraries that support data manipulation, analysis, and visualization.
- Applications: Data cleaning, statistical analysis, machine learning, and data visualization.
4) SPSS (Statistical Package for the Social Sciences):
- Description: A comprehensive statistical software suite used for data analysis, data mining, and predictive analytics.
- Applications: Descriptive statistics, hypothesis testing, regression analysis, and advanced analytics.
5) SAS (Statistical Analysis System):
- Description: A software suite used for advanced analytics, multivariate analysis, and predictive modeling.
- Applications: Data management, statistical analysis, predictive modeling, and business intelligence.
6) Tableau:
- Description: A data visualization tool that allows users to create interactive and shareable dashboards and reports.
- Applications: Data visualization , business intelligence , and interactive dashboard creation.
7) Power BI:
- Description: A business analytics tool developed by Microsoft that provides interactive visualizations and business intelligence capabilities.
- Applications: Data visualization, business intelligence, reporting, and dashboard creation.
8) SQL (Structured Query Language) Databases (e.g., MySQL, PostgreSQL, Microsoft SQL Server):
- Description: Database management systems that support data storage, retrieval, and manipulation using SQL queries.
- Applications: Data retrieval, data cleaning, data transformation, and database management.
9) Apache Spark:
- Description: A fast and general-purpose distributed computing system designed for big data processing and analytics.
- Applications: Big data processing, machine learning, data streaming, and real-time analytics.
10) IBM SPSS Modeler:
- Description: A data mining software application used for building predictive models and conducting advanced analytics.
- Applications: Predictive modeling, data mining, statistical analysis, and decision optimization.
These tools serve various purposes and cater to different data analysis needs, from basic statistical analysis and data visualization to advanced analytics, machine learning, and big data processing. The choice of a specific tool often depends on the nature of the data, the complexity of the analysis, and the specific requirements of the project or organization.
Also Read: How to Analyze Survey Data: Methods & Examples
Importance of Data Analysis in Research
The importance of data analysis in research cannot be overstated; it serves as the backbone of any scientific investigation or study. Here are several key reasons why data analysis is crucial in the research process:
- Data analysis helps ensure that the results obtained are valid and reliable. By systematically examining the data, researchers can identify any inconsistencies or anomalies that may affect the credibility of the findings.
- Effective data analysis provides researchers with the necessary information to make informed decisions. By interpreting the collected data, researchers can draw conclusions, make predictions, or formulate recommendations based on evidence rather than intuition or guesswork.
- Data analysis allows researchers to identify patterns, trends, and relationships within the data. This can lead to a deeper understanding of the research topic, enabling researchers to uncover insights that may not be immediately apparent.
- In empirical research, data analysis plays a critical role in testing hypotheses. Researchers collect data to either support or refute their hypotheses, and data analysis provides the tools and techniques to evaluate these hypotheses rigorously.
- Transparent and well-executed data analysis enhances the credibility of research findings. By clearly documenting the data analysis methods and procedures, researchers allow others to replicate the study, thereby contributing to the reproducibility of research findings.
- In fields such as business or healthcare, data analysis helps organizations allocate resources more efficiently. By analyzing data on consumer behavior, market trends, or patient outcomes, organizations can make strategic decisions about resource allocation, budgeting, and planning.
- In public policy and social sciences, data analysis is instrumental in developing and evaluating policies and interventions. By analyzing data on social, economic, or environmental factors, policymakers can assess the effectiveness of existing policies and inform the development of new ones.
- Data analysis allows for continuous improvement in research methods and practices. By analyzing past research projects, identifying areas for improvement, and implementing changes based on data-driven insights, researchers can refine their approaches and enhance the quality of future research endeavors.
However, it is important to remember that mastering these techniques requires practice and continuous learning. That’s why we highly recommend the Data Analytics Course by Physics Wallah . Not only does it cover all the fundamentals of data analysis, but it also provides hands-on experience with various tools such as Excel, Python, and Tableau. Plus, if you use the “ READER ” coupon code at checkout, you can get a special discount on the course.
For Latest Tech Related Information, Join Our Official Free Telegram Group : PW Skills Telegram Group
Data Analysis Techniques in Research FAQs
What are the 5 techniques for data analysis.
The five techniques for data analysis include: Descriptive Analysis Diagnostic Analysis Predictive Analysis Prescriptive Analysis Qualitative Analysis
What are techniques of data analysis in research?
Techniques of data analysis in research encompass both qualitative and quantitative methods. These techniques involve processes like summarizing raw data, investigating causes of events, forecasting future outcomes, offering recommendations based on predictions, and examining non-numerical data to understand concepts or experiences.
What are the 3 methods of data analysis?
The three primary methods of data analysis are: Qualitative Analysis Quantitative Analysis Mixed-Methods Analysis
What are the four types of data analysis techniques?
The four types of data analysis techniques are: Descriptive Analysis Diagnostic Analysis Predictive Analysis Prescriptive Analysis
- Top 10 Data Analytics Courses
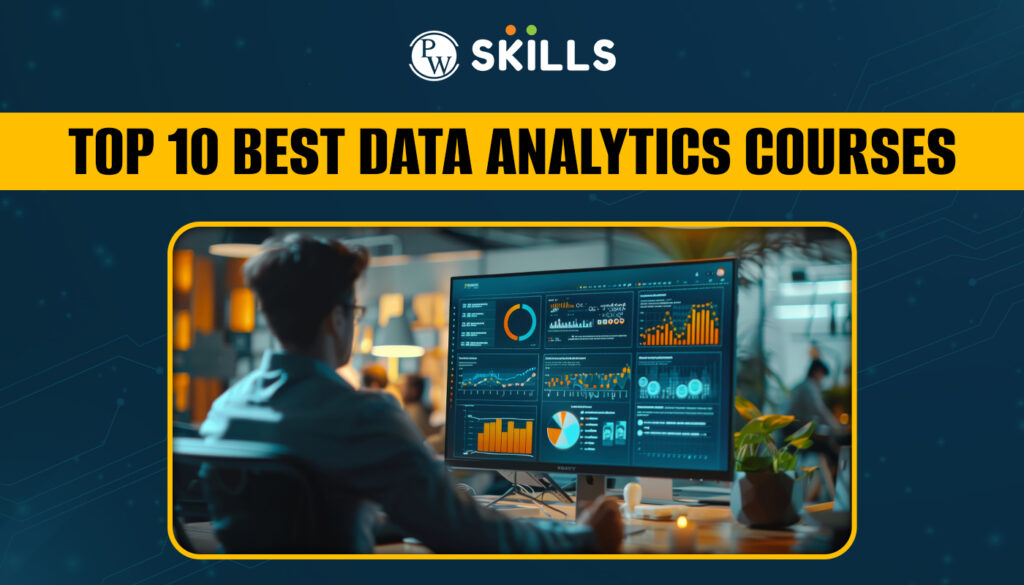
Top 10 Best Data Analytics Courses to boost your career in 2025. Explore top online programs, certifications, and resources to…
- Business Intelligence Programs – Top 15 Business Intelligence Tools in 2025
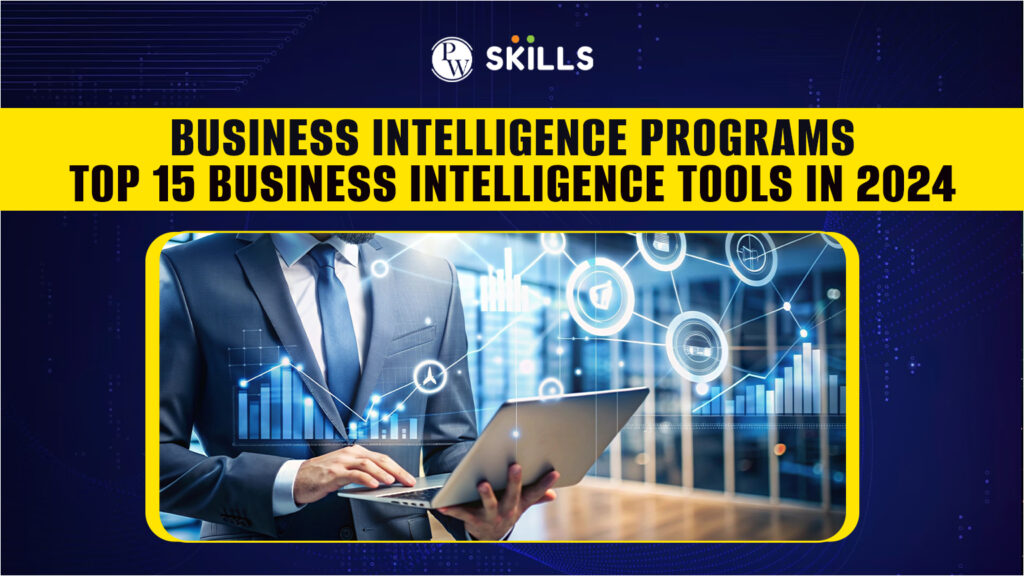
Business Intelligence Programs empower organizations to transform data into actionable insights, enhancing decision-making and driving operational efficiency. Checkout the Top…
- What is Statistical Modeling? Definition and FAQs
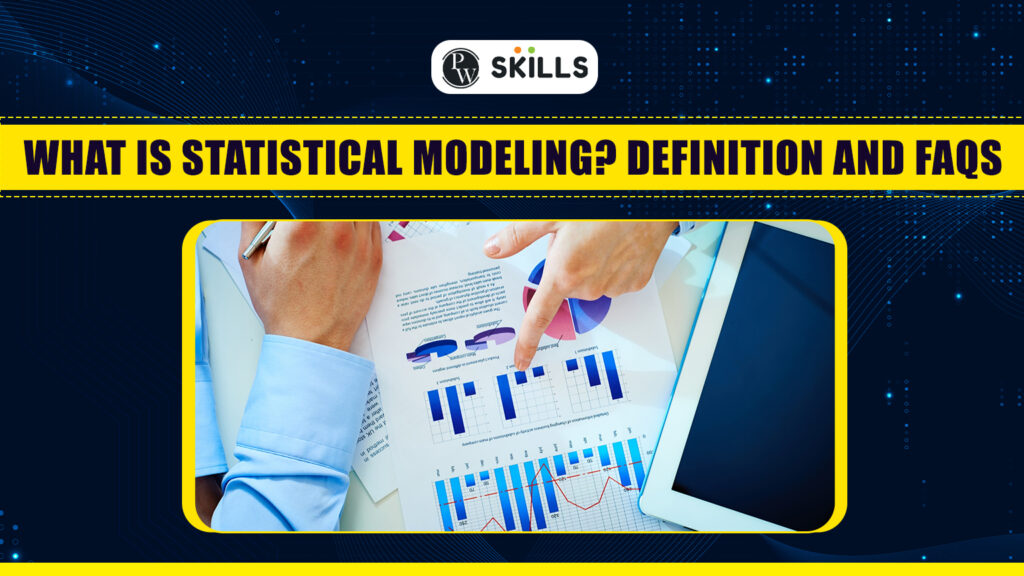
Statistical Modeling states scover the definition, key concepts, and frequently asked questions about statistical modeling, including its applications in data…
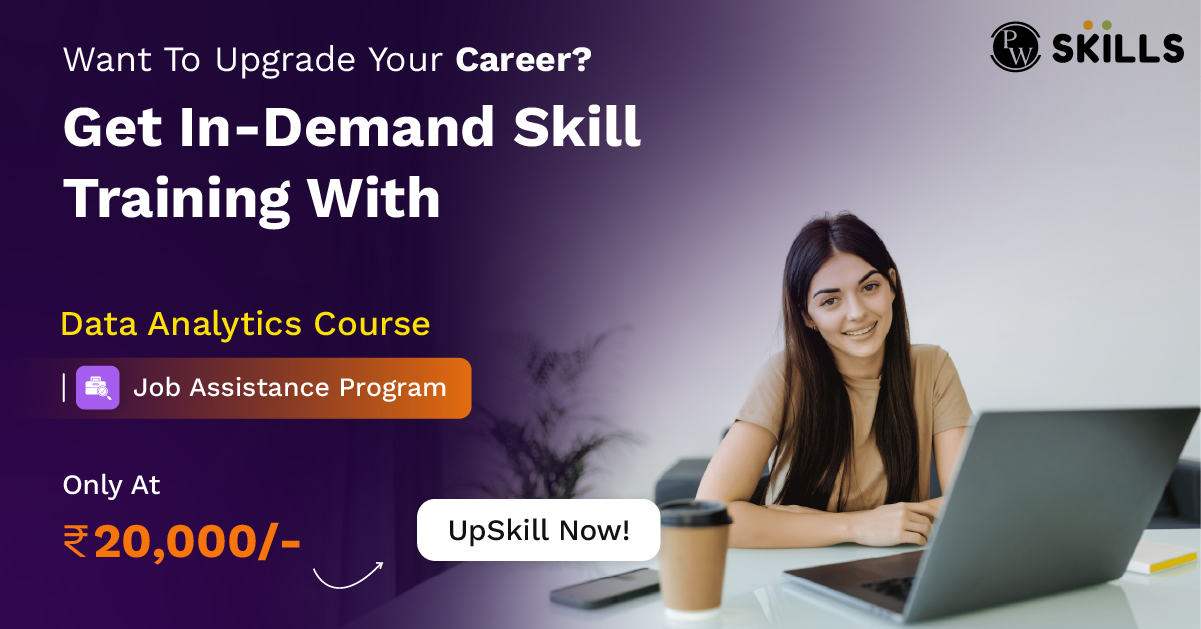
Related Articles
- What is Diagnostic Analytics? Why it is Important
- Data Analyst Recruitment For Fresher By S&P Global, Apply Now!
- Cluster Analysis – Methods, Applications, and Algorithms
- Top 5 Data Analytics Jobs For Freshers In October
- 5 Examples Of Descriptive Analytics
- What Is Retail Analytics? The Ultimate Guide
- What Is Lexical Analysis? Explore In Detail
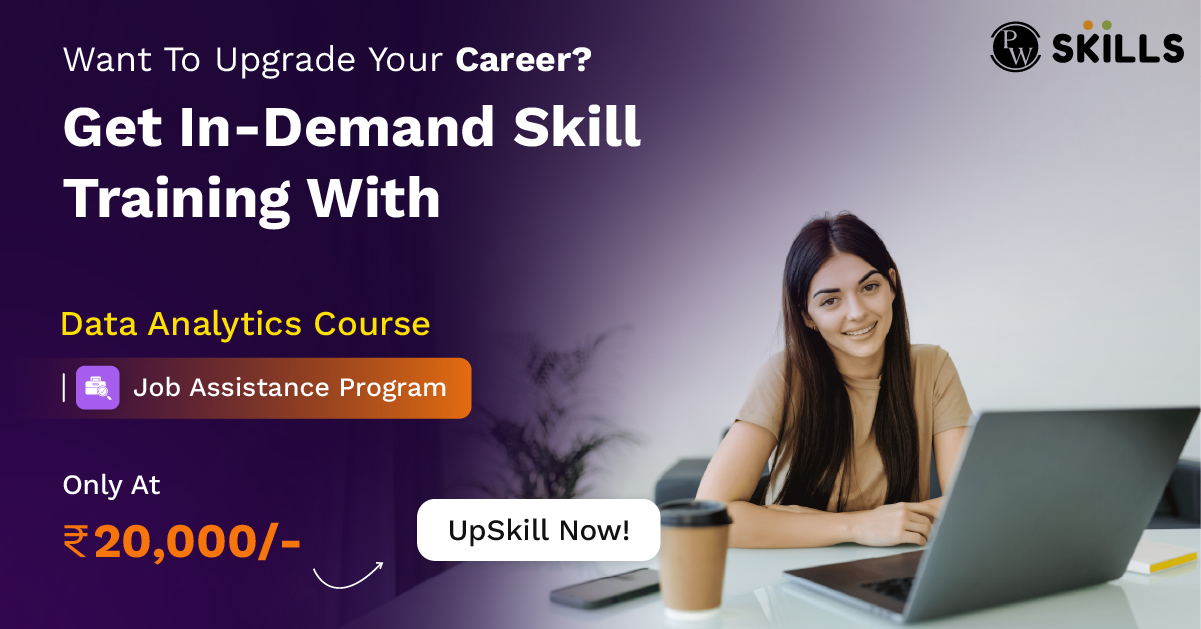

IMAGES
VIDEO
COMMENTS
Research analysis is a critical component of the research process, serving as the bridge between data collection and the extraction of meaningful insights. It involves evaluating and interpreting the information gathered during research to make informed decisions and conclusions. This process is not merely about presenting data; it requires a ...
Research analytics fosters a collaborative environment among research teams by providing tools and platforms that facilitate communication and knowledge sharing. Analytics tools often feature collaborative functionalities, allowing team members to work together in real-time, share insights, and provide feedback. ...
The Research Analytics (RA) team in the Division of Research, Innovation & Impact provides research and sponsored projects reporting, analyses and strategic decision support for the MU community. Our work products can be categorized into: Routine reporting that is accurate, insightful and timely.
Evaluate the outcomes and effectiveness of an innovative public health program on community well-being. Applying psychometric methods to improve the reliability and validity of accreditation processes. Over 20 Years of Research Analysis Consulting Experience. Help achieve your research analytic goals with our team of experts in measurement and ...
Definition of research in data analysis: According to LeCompte and Schensul, research data analysis is a process used by researchers to reduce data to a story and interpret it to derive insights. The data analysis process helps reduce a large chunk of data into smaller fragments, which makes sense. Three essential things occur during the data ...
Data analysis is the systematic process of inspecting, cleaning, transforming, and modeling data to uncover meaningful insights, support decision-making, and solve specific problems. In today's data-driven world, data analysis is crucial for businesses, researchers, and policymakers to interpret trends, predict outcomes, and make informed decisions.
Learn how to choose and use research methods for collecting and analyzing data. Compare qualitative and quantitative, primary and secondary, descriptive and experimental methods with examples and pros and cons.
Data Analysis Techniques in Research: While various groups, institutions, and professionals may have diverse approaches to data analysis, a universal definition captures its essence.Data analysis involves refining, transforming, and interpreting raw data to derive actionable insights that guide informed decision-making for businesses.
Statistical analysis means investigating trends, patterns, and relationships using quantitative data. It is an important research tool used by scientists, governments, businesses, and other organizations. To draw valid conclusions, statistical analysis requires careful planning from the very start of the research process. You need to specify ...
data analysis, the process of systematically collecting, cleaning, transforming, describing, modeling, and interpreting data, generally employing statistical techniques. Data analysis is an important part of both scientific research and business, where demand has grown in recent years for data-driven decision making.Data analysis techniques are used to gain useful insights from datasets, which ...